IIIT-AR-13K: A New Dataset for Graphical Object Detection in Documents
Title: IIIT-AR-13K: A New Dataset for Graphical Object Detection in Documents
Authors : Ajoy Mondal, Peter Lipps and C. V. Jawahar
Abstract
We introduce a new dataset for graphical object detection in business documents, more specifically annual reports. This dataset, IIIT-AR-13K, is created by manually annotating the bounding boxes of graphical or page objects in publicly available annual reports. This dataset contains a total of 13K annotated page images with objects in five different popular categories — table, figure, natural image, logo, and signature. This is the largest manually annotated dataset for graphical object detection. Annual reports created in multiple languages for several years from various companies bring high diversity into this dataset. We benchmark IIIT-AR-13K dataset with two state of the art graphical object detection techniques using Faster R-CNN [18] and Mask R-CNN [11] and establish high baselines for further research. Our dataset is highly effective as training data for developing practical solutions for graphical object detection in both business documents and technical articles. By training with IIIT-AR-13K, we demonstrate the feasibility of a single solution that can report superior performance compared to the equivalent ones trained with a much larger amount of data, for table detection. We hope that our dataset helps in advancing the research for detecting various types of graphical objects in business documents.
Keywords: graphical object detection · annual reports · business documents · Faster R-CNN · Mask R-CNN.
Dataset: IIIT-AR-13K
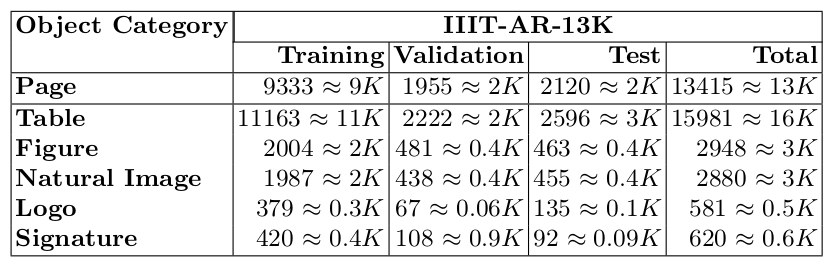
Table 1: Statistics of our newly generated IIIT-AR-13K dataset.
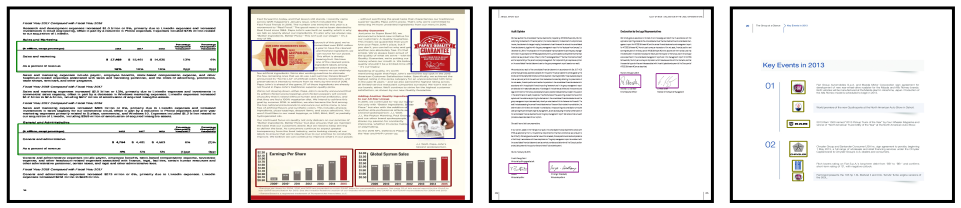
Fig. 1: Sample annotated document images of IIIT-AR-13K dataset. Dark Green: indicates ground truth bounding box of table, Dark Red: indicates ground truth bounding box of figure, Dark Blue: indicates ground truth bound- ing box of natural image, Dark Yellow: indicates ground truth bounding box of logo and Dark Pink: indicates ground truth bounding box of signature.
Comparison with the Existing Datasets
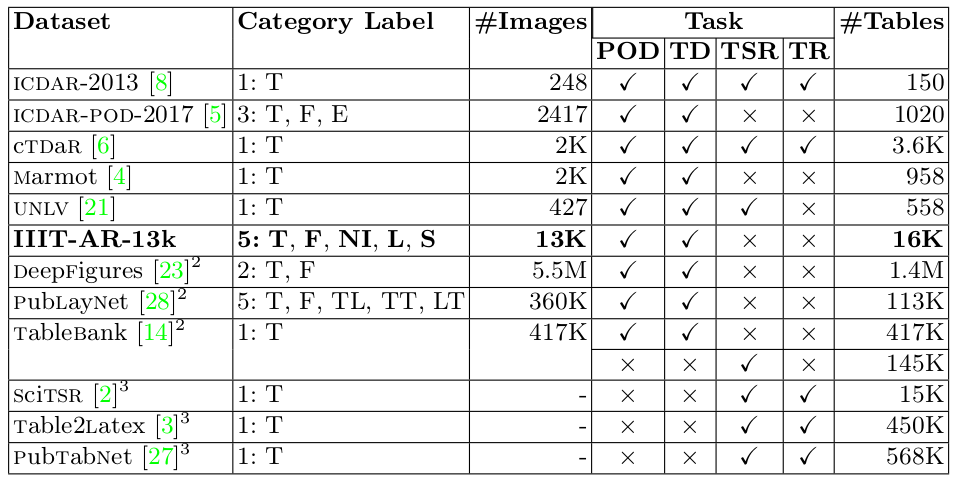
Table 2: Statistics of existing datasets along with newly generated dataset for graphical object detection task in document images. T: indicates table. F: indicates figure. E: indicates equation. NI: indicates natural image. L: indicates logo. S: indicates signature. TL: indicates title. TT: indicates text. LT: indicates list. POD: indicates page object detection. TD: indicates table detection. TSR: indicates table structure recognition. TR: indicates table recognition.
Diversity in Object Category
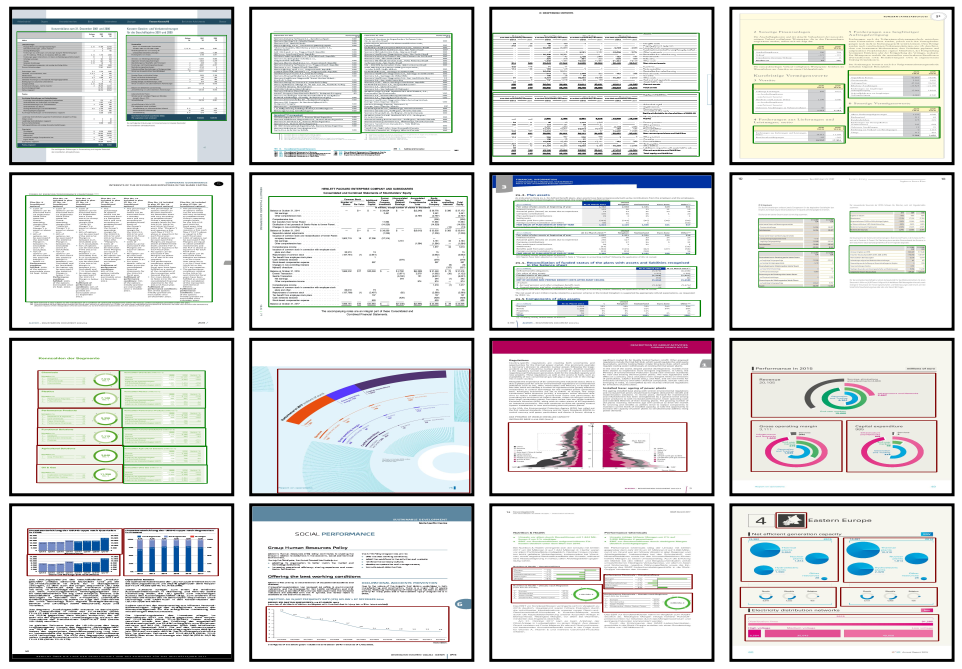
Fig. 2: Sample annotated images with large variation in tables and figures.
Performance for Detection using Baseline Methods
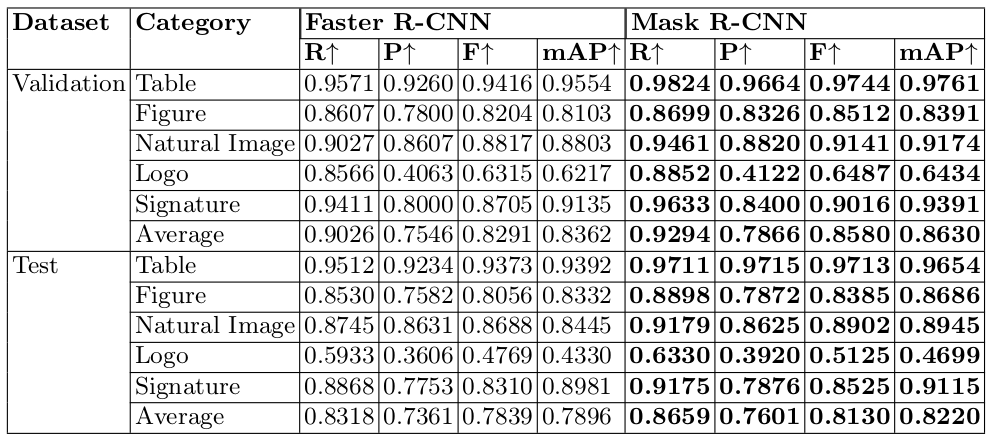
Table 3: Quantitative results of two baseline approaches for detecting graphical objects. R: indicates Recall. P: indicates Precision. F: indicates f-measure. mAP: indicates mean average precision.
Effectiveness of IIIT-AR-13K Over Existing Larger Datasets
- Experiment-I: Mask R-CNN trained with Tablebank (Latex) dataset.
- Experiment-II: Mask R-CNN trained with Tablebank (Word) dataset.
- Experiment-III: Mask R-CNN trained with Tablebank (Latex+Word) dataset.
- Experiment-IV: Mask R-CNN trained with PubLayNet dataset.
- Experiment-V: mask R-CNN trained with IIIT-AR-13K dataset.
Observation-I - Without Fine-tuning:
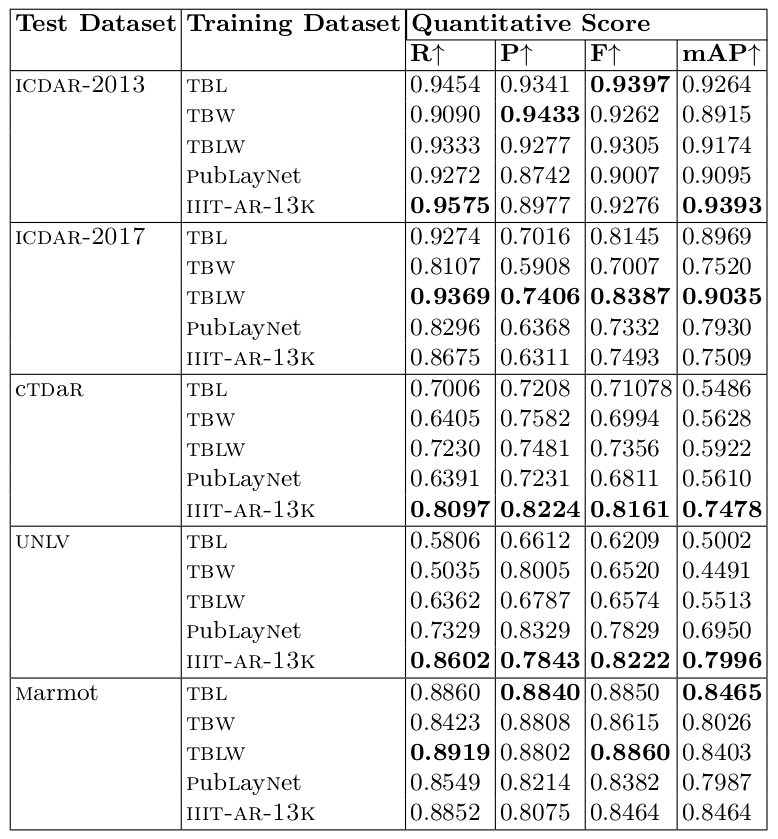
Table 4: Performance of table detection in the existing datasets. Model is trained with only training images containing tables of the respective datasets. TBL: indicates tablebank (latex). TBW: indicates tablebank (word). TBLW: indicates tablebank (latex+word). R: indicates Recall. P: indicates Precision. F: indicates f-measure. mAP: indicates mean average precision.
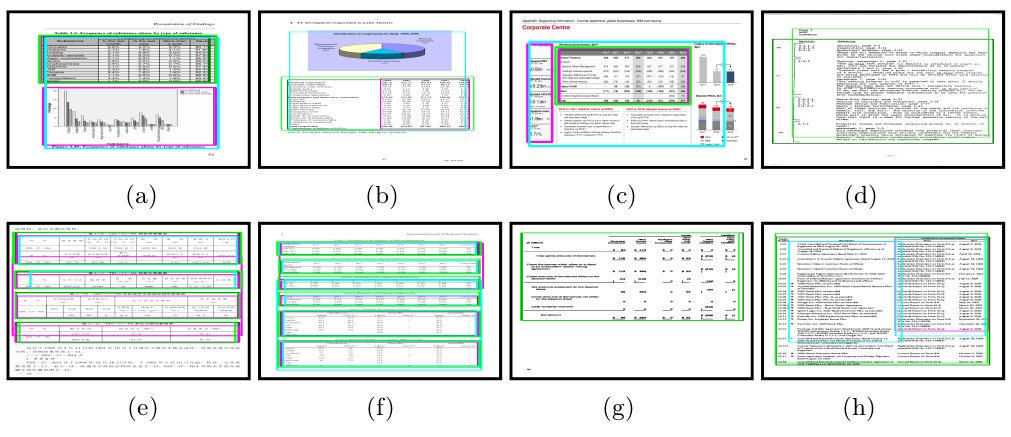
Fig. 3: Few examples of predicted bounding boxes of tables in various datasets - (a) ICDAR-2013, (b) ICDAR-POD-2017, (c) cTDaR, (d) UNLV, (e) Marmot, (f) PubLayNet, (g) IIIT-AR-13K (validation), (h) IIIT-AR-13K (test) using Experiment-I, Experiment-IV and Experiment-V. Experiment-I: Mask R-CNN trained with tablebank (latex) dataset. Experiment-IV: Mask R-CNN trained with PubLayNet dataset. Experiment-V: Mask R-CNN trained with IIIT-AR-13K dataset. Dark Green: rectangle highlights the ground truth bounding boxes of tables. Pink, Cyne and Light Green: rectangles indicate the predicted bounding boxes of tables using Experiment-I, Experiment-IV and Experiment-V, respectively.
Observation-II - Fine-tuning with Complete Training Set and Observation-III - Fine-tuning with Partial Training Set
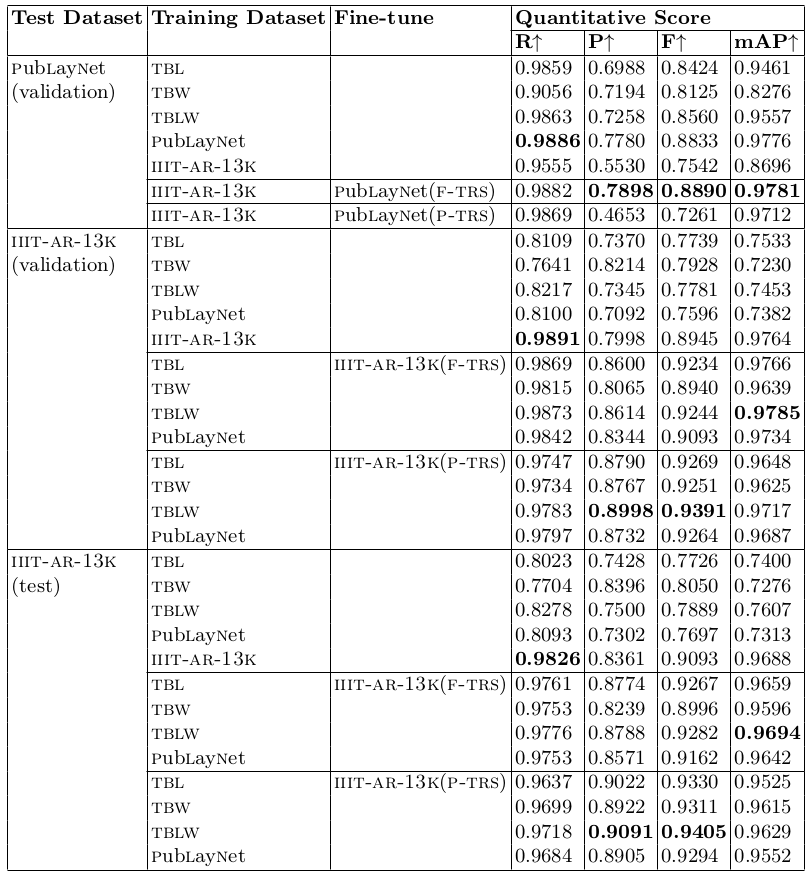
Table 5: Performance of table detection using fine-tuning. TBL: indicates tablebank (latex). TBW: indicates tablebank (word). TBLW: indicates tablebank (latex+word). F-TRS: indicates complete training set. P-TRS: indicates partial training set i.e. only 1k randomly selected training images. R: indicates Recall. P: indicates Precision. F: indicates f-measure. mAP: indicates mean average precision.
Link to the Download: [ Dataset ] , [ Code ] & [ PDF ]
Publication
- Ajoy Mondal, Peter Lipps, and C. V. Jawahar , IIIT-AR-13K: A New Dataset for Graphical Object Detection in Documents , In Proceeding of International Workshop on Document Analysis Systems 2020.
Cite this paper as:
Mondal A., Lipps P., Jawahar C.V. (2020) IIIT-AR-13K: A New Dataset for Graphical Object Detection in Documents. In: Bai X., Karatzas D., Lopresti D. (eds) Document Analysis Systems. DAS 2020. Lecture Notes in Computer Science, vol 12116. Springer, Cham. https://doi.org/10.1007/978-3-030-57058-3_16
Team
OpenText
- Peter Lipps
- Isaac Rajkumar
- Sreelatha Samala
- Sangeetha Yanamandra
CVIT, IIIT, Hyderabad
- Prof. C. V. Jawahar
- Dr. Ajoy Mondal
- Sachin Raja
- K. V. Jobin
- Boddapati Mahesh
- Madhav Agarwal
Acknowledgment
We are thankful to the staff, annotation team of CVIT, and OpenText for their support.