Indic Scene Text on the Roadside
Title: Indic Scene Text on the Roadside
Authors : Ajoy Mondal ,Krishna Tulsyan and C V Jawahar
Abstract
Extensive research and the development of benchmark datasets have primarily
focused on Scene Text Recognition (STR) in Latin languages. However, the scenario differs for
Indian languages, where the complexities in syntax and semantics have posed many challenges,
resulting in limited datasets and comparatively less research in this domain. Overcoming
these challenges is crucial for advancing scene text recognition in Indian languages. Although
a few works have touched upon this issue, they are constrained in the size and scale of
the data as far as we know. To bridge this gap, this paper introduces a large scale, diverse
dataset, named as IIIT-IndicSTR-Word for Indic scene text. Comprising a total of 250K word
level images in ten different languages — Bengali, Gujarati, Hindi, Kannada, Malayalam,
Marathi, Odia, Punjabi, Tamil, and Telugu, these images are extracted from roadside scenes
captured by a GoPro camera. The dataset encompasses a wide array of realistic adversarial
conditions, including blur, changes in illumination, occlusion, non-iconic texts, low resolution,
and perspective text. We establish a baseline for the proposed dataset, facilitating evaluation
and benchmarking with a specific focus on STR tasks. Our findings indicate that our dataset is
a practical training source to enhance performance on respective datasets. The code, dataset,
and benchmark results are available at https://cvit.iiit.ac.in/usodi/istr.php.
Keywords: Scene Text Recognition (STR). word images. Indic languages. Indic scene text.
roadside. benchmark·
Introduction
Language serves as a universal medium for global communication, facilitating exchanges and interactions among people worldwide. The diverse array of languages across different communities highlights the recognition of language’s crucial role in human connectivity. As a written form of language, text significantly enhances the potential for information transfer. In the wild, where writing manifests semantic richness, valuable information that holds the key to understanding the contemporary environment is embedded. The textual information found in diverse settings is pivotal in various applications, ranging from image search and translation to transliteration, assistive technologies (especially for the visually impaired), and autonomous navigation. In the modern era, the automatic extraction of text from photographs or frames depicting natural environments, known as Scene Text Recognition (STR) or Photo-OCR, is a significant challenge. This complex problem is typically divided into two sub-problems: scene text detection, which involves locating text within an image, and cropped word image recognition.
Optical Character Recognition (OCR) has traditionally concentrated on interpreting printed or handwritten text within documents. However, the proliferation of capturing devices like mobile
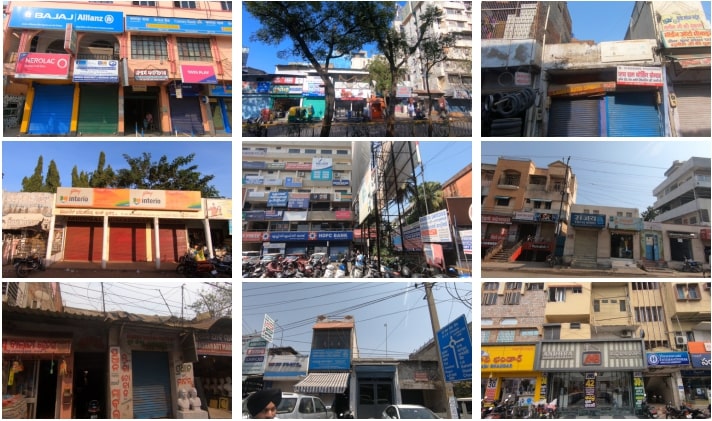
Fig. 1.Showcases valuable sources of scene text in various Indic languages within roadside images captured by a camera.
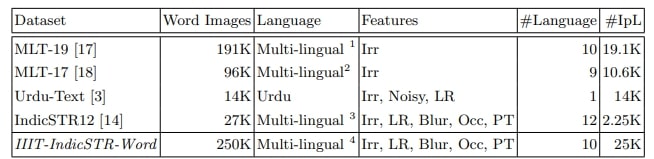
Table 1. Statistics regarding several publicly available real scene text recognition datasets are presented. #Language denotes the total number of languages in each dataset, while #IpL represents the average number of word level images per language. Irr, LR, Occ, and PT indicate the presence of irregular text, low resolution images, occlusion, and perspective text, respectively.
phones and video cameras has underscored the significance of scene text recognition (STR), presenting a challenge whose resolution holds substantial potential for advancing various applications.
Despite the heightened interest in STR within the research community, it comes with unique challenges, including varying backgrounds in natural scenes, diverse scripts, fonts, layouts, styles, and image imperfections related to text, such as blurriness, occlusion, and uneven illumination.
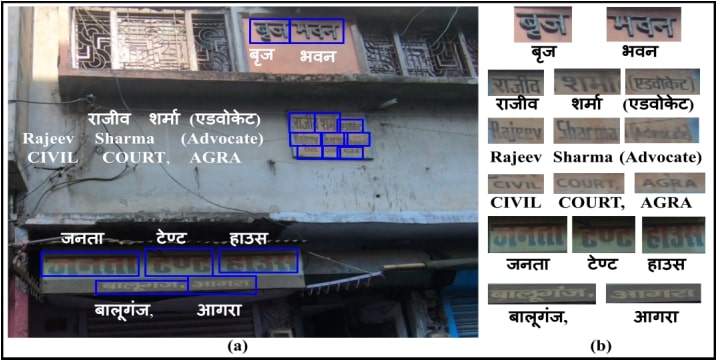
Fig. 2. (a) Depicts a single annotated image frame from our dataset. (b) Represents cropped word level images and their corresponding transcriptions serve as ground truths.
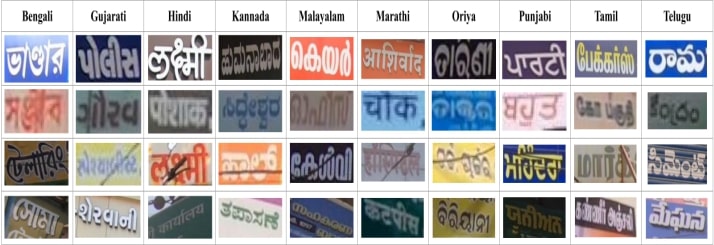
Fig. 3. Illustrates a few sample word level images of each of the ten languages from our dataset.
In the context of Indic languages, relatively few efforts have focused on datasets and models. For instance, MLT-19 [17] (featuring only Bengali and Devanagari), MLT-17 [18] (limited to Bengali), Urdu-Text [3], and IndicSTR12 [14] (covering twelve Indic languages) are some examples. IndicSTR12 is the largest Indic scene text recognition dataset, encompassing twelve languages spoken in India. However, it contains a limited number of word level images (approximately 2K-3K) per language, which needs to be increased for training deep STR models. Consequently, there is a growing need for a more extensive and diverse dataset explicitly designed for STR in Indic languages. This demand has emerged to meet the evolving research requirements in scene text recognition for Indic scripts.
To bridge this gap, our paper introduces a large scale, diverse dataset, named as IIIT-IndicSTRWord for scene text recognition in Indic languages. Roadside scene images are a rich resource of scene text images for STR tasks. Illustrated in Fig. 1 are Indian roadside scene images captured across different states. These images exhibit diverse text characteristics, including occlusion or partial occlusion, variations in illumination, motion blur, perspective distortion, orientation differences, and variations in style, size, color, and language. They serve as invaluable assets for constructing expansive Indic scene text recognition datasets. Leveraging this resource, we meticulously capture numerous roadside scene images from diverse Indian states using a GoPro camera. Subsequently, we extract words from these images and annotate them with corresponding transcriptions, which serve as ground truths 5 (refer Fig. 2). The resulting dataset encompasses 250K word level images spanning ten languages: Bengali, Gujarati, Hindi, Kannada, Malayalam, Marathi, Oriya, Punjabi, Tamil, and Telugu. Additionally, we present a high-performing baseline for STR in Indic languages. In summary, our contributions can be outlined as follows:
- We present IIIT-IndicSTR-Word, a vast and diverse Indic scene text recognition dataset. It comprises 250K word level images extracted from roadside scenes captured by GoPro cameras. Encompassing ten major languages of India — Bengali, Gujarati, Hindi, Kannada, Malayalam, Oriya, Punjabi, Tamil, and Telugu (refer to Fig. 3), our dataset provides comprehensive coverage. For a thorough overview and comparison with existing datasets, please consult Table 1. The statistics demonstrate that our dataset offers remarkable diversity and is notably larger than existing Indic STR datasets. To our knowledge, it is the most extensive dataset for Indic scene text recognition.
- The images in our dataset, encompass a wide range of variations, including differences in font styles, low resolution, partial occlusion, perspective imaging, illumination variations, varying text lengths, and multi-orientation across languages (refer Fig. 4). This diverse collection is pivotal in developing robust and high-performing Indic scene text recognition models.
- We offer a baseline model for the scene text recognition task. The results showcase how the dataset enhances the performance of the model (refer Table 4 and Table 5). Our findings suggest that our dataset is a valuable training resource to enhance performance on corresponding datasets.
IIIT-IndicSTR-Word Dataset
We gather several thousand diverse roadside scene images using GoPro cameras from two to three metropolitan cities across various states of India. Primarily we cover states — West Bengal, Gujarat, Delhi, Karnataka, Kerala, Maharashtra, Odisha, Punjab, Tamil Nadu, and Telangana to capture scene text images of corresponding languages — Bengali, Gujarati, Hindi, Kannada, Malayalam, Oriya, Punjabi, Tamil, and Telugu. We capture images during day times. Subsequently, we extract word images from these scene images and manually generate transcripts for these word images as ground truth data for the text recognition task. This process leads to the creation of data corpus,
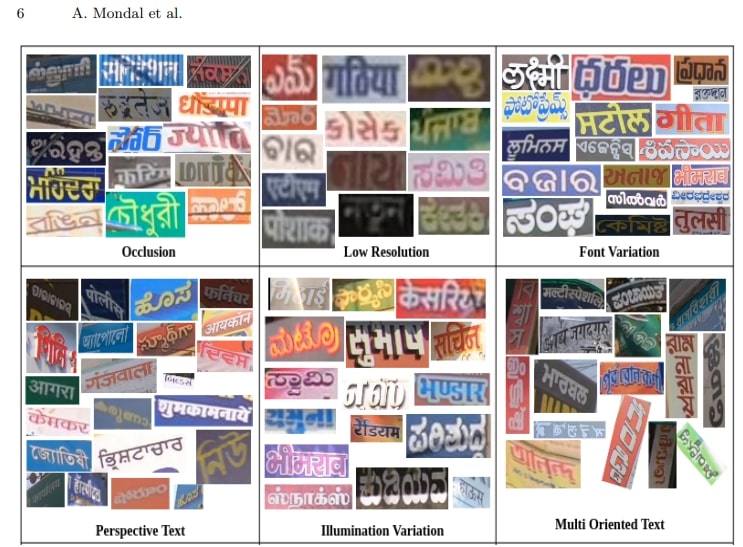
Fig. 4. The images showcased in this display exhibit various word samples sourced from our dataset. These words are extracted from roadside scene images captured by a GoPro camera. The collection encompasses a variety of word images, showcasing features such as partial occlusion, low resolution, font variation, perspective text, illumination variation, and multi oriented texts.
comprising 250K word level images representing ten popular Indic languages: Bengali, Gujarati, Hindi, Kannada, Malayalam, Oriya, Punjabi, Tamil, and Telugu. To represent the ground truth data corpus, we utilize the standard XML format, which includes the names of word level images and their corresponding textual transcriptions. Fig. 2 presents a sample annotation for reference. In Fig. 2(a), depicts a single annotated image frame from our dataset, while Fig. 2 represents cropped word level images, and their corresponding transcriptions serve as ground truths.
3.1 Dataset Feature and Statistics
Diversity: As scene text images in ten distinct languages are captured from various states of India, the resulting dataset is remarkably diverse. Fig. 4 provides a glimpse of this diversity through a selection of sample word images spanning multiple languages from our dataset. These images encompass a wide range of variations, including differences in font styles, low resolution, partial occlusion, perspective imaging, illumination variations, varying text lengths, and multi orientation across languages. This diverse collection is pivotal in developing robust and high-performing Indic Indic scene text recognition models. By encompassing such a broad spectrum of linguistic and visual characteristics, the dataset serves as a valuable resource for advancing research in this field and enhancing the accuracy and versatility of scene text recognition systems.
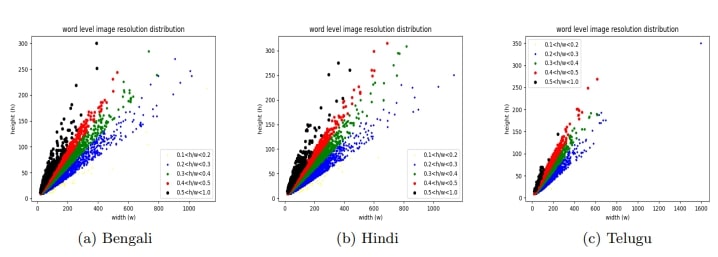
Fig. 5. Shows word level image resolution distribution for three languages Bengali, Hindi and Telugu.
Word Level Image Resolution Distribution: The variation in language, font, and geographical regions across India contributes to the diversity in the resolution of scene text words. This diversity in word resolution significantly improves the model’s ability to generalize. Fig. 5 illustrates the distribution of resolutions in word level images, showcasing the dataset’s extensive variability. It is evident from the figure that the resolution of word level images varies across different languages6 .Incorporating words with diverse resolutions enriches the dataset, allowing the model to accommodate a broader range of visual characteristics and enhancing its performance across various styles and imaging conditions.
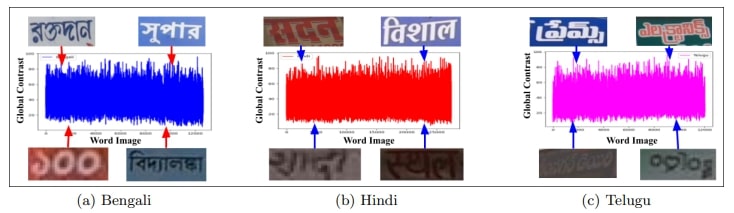
Fig. 6. Shows varying global contrast among word images in our dataset for three languages Bengali, Hindi and Telugu.
Contrast of Word Images: As word images are extracted from roadside scene images captured by GoPro cameras, the intensities vary significantly from one word image to another, resulting in fluctuations in contrast. To assess the ease of recognition for each word image, we employ the global contrast strategy [10]. Fig. 6 showcases the diverse global contrast levels among word images in Bengali, Hindi, and Telugu languages, respectively. The figures demonstrate that contrast levels vary between 20 and 70 across the three languages. This variability in intensity within word images increases the complexity of the dataset and contributes to the creation of robust STR models. In contrast, understanding and leveraging these variations can enhance the adaptability and effectiveness of STR algorithms across diverse linguistic contexts. Additionally, exploring similar analyses for other languages included in the dataset can offer further insights into its characteristics and implications for STR model performance.
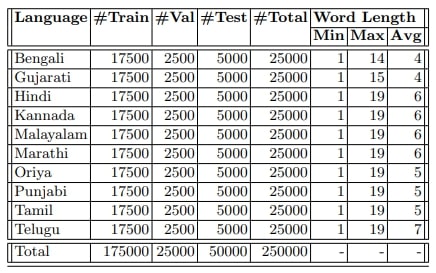
Table 2. The breakdown of our dataset into training, validation, and testing sets is presented for each language.
Dataset Split: Due to limitation of real Indic STR datasets, synthetic scene text [14] plays an important role for pre-training deep architecture. However, limited real scene text images are not able to reduce the domain gap between real and synthetic scene text images, resulting in poor recognition accuracy in real situation. To keep in mind, we divide our dataset containing 250K word images into 175K word images for training, 25K word images for validation, and 50K word images for testing. Table 2 shows statistics of our dataset. It will ensure that the models are trained on a large and diverse dataset and will help to improve their accuracy and performance.
3.2 Comparison with Existing Datasets
Table 3 compares our dataset and existing Latin, multi-lingual, and Indic scene text recognition datasets, highlighting significant differences and advantages. Various factors, such as dataset size and diversity in word level images — encompassing partial occlusion, font variation, illumination variation, perspective text, multi-oriented text, and text of varying lengths — are meticulously evaluated.
Compared to the current IndicSTR12 dataset, our proposed dataset, is six times larger, resulting in a more extensive collection of unique scene text word images. Additionally, our dataset IIITIndicSTR-word surpasses existing multi-lingual STR datasets, 1.3 times larger than MLT-19, 2.6 times larger than MLT-17 and 5 times larger than LSVT. It also exceeds the size of existing Latin STR datasets, including IIIT5K-Words, SVT, ICDAR2003, ICDAR2013, ICDAR2015, and SVT Perspective. Our dataset with 250K word images, compares favorably with existing multi-lingual dataset such as MTWI (289K word images). Furthermore, our experimental section explores the potential impact of these differences on the performance and generalization capabilities of models trained on each dataset. This comparative analysis aims to provide a comprehensive understanding of our proposed dataset’s distinctive contributions and characteristics within the broader context of existing resources, offering valuable insights for researchers and practitioners alike.
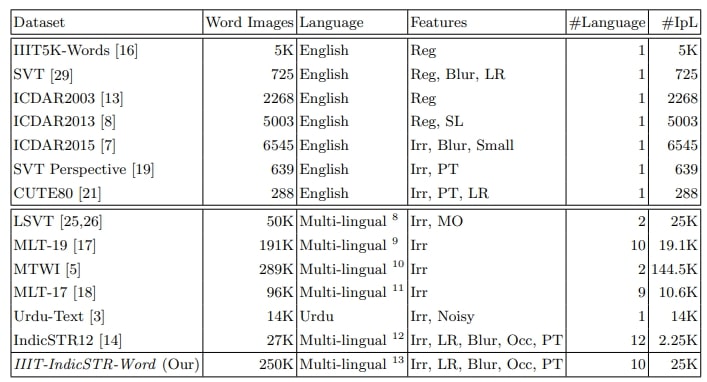
Table 3. Illustrate comparison of our dataset with various public real scene text recognition datasets. #Language denotes the total number of languages in each dataset, while #IpL represents the average number of word level images per language. Reg, Irr, LR, Occ, Mo and PT, indicate the presence of regular text, irregular text, low resolution images, occlusion, multi oriented text and perspective text, respectively
4.2 Benchmark Results on Word Recognition - Performance on Our Dataset:
Performance on Our Dataset: We utilize synthetic images specific to each language sourced from the IndicSTR12 dataset [14] to pre-train the PARSeq model. Subsequently, we train the PARSeq model using images from the training set of our dataset, followed by an evaluation of the
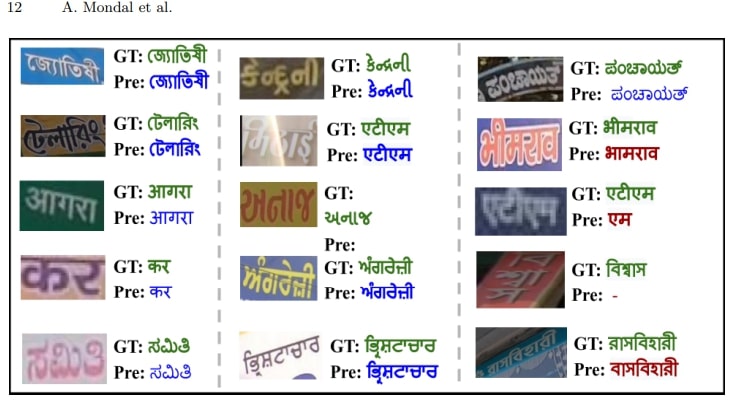
Fig. 7. Present a few sample visual results on test sets of our dataset. Green, Blue, and Red colored text indicate ground truth, correct prediction, and wrong prediction, respectively.
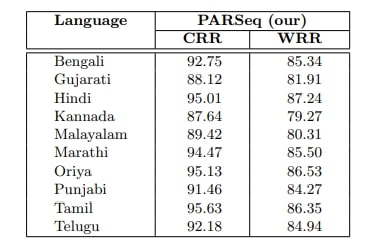
Table 4. Performance of the baseline PARSeq models on test sets of our dataset.
respective test sets for each language. Separate PARSeq models are trained for each language. The results obtained on the test sets of our dataset are presented in Table 4. The table underscores that pre-training the PARSeq model with synthetic images from the IndicSTR12 dataset and then training it with real images from our dataset enhances accuracy in terms of CRR and WRR, owing to the large number of images present in the training set of our dataset. A more extensive training set contributes to improved model performance.
Fig. 7 displays select visual outcomes derived from the test sets of our dataset. The illustrations reveal that PARSeq effectively identifies standard text segments. However, when confronted with multi-oriented or low resolution text, PARSeq struggles to recognize the text accurately.
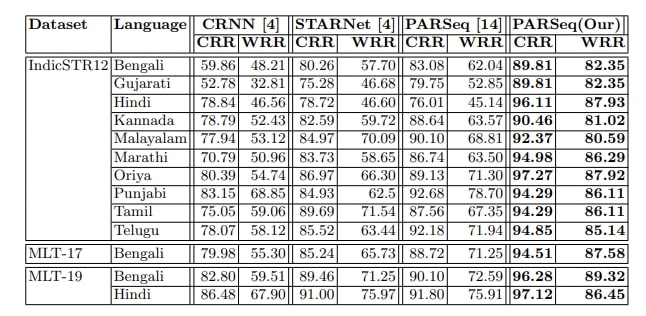
Table 5. Performance of the baseline PARSeq models on existing Indic STR datasets.
Conclusions
We introduce IIIT-IndicSTR-Word, a comprehensive and diverse collection tailored for Indic scene text recognition tasks. Comprising 250K word level images across ten different languages (Bengali, Gujarati, Hindi, Kannada, Malayalam, Marathi, Oriya, Punjabi, Tamil, and Telugu), these images are sourced from roadside scenes captured via a GoPro camera. Notably, the dataset encompasses a broad spectrum of real-world scenarios, including variations in blur, lighting conditions, occlusion, non-standard text orientations, low image resolutions, and perspective distortions. Our study presents benchmark results achieved by applying established architecture for text recognition tasks. Additionally, our experiments demonstrate that training model using our dataset leads to notable improvements in model performance.
Future research avenues could explore end-to-end approaches that integrate text localization and recognition within a unified framework. We eagerly welcome contributions from researchers and developers interested in leveraging this dataset to develop new models and advance the field of Indic scene text recognition.
Link to the Download: [ Code ] & [ pdf ]
Datasets:
[ Bengali ] , [ Gujarati ] , [ Hindi ] , [Kannada ] , [ Malayalam ] , [Marathi ] , [ Oriya ] , [Punjabi ] , [ Tamil ], [ Telugu ]
Publication
- Ajoy Mondal, Krishna Tulsyan and C V Jawahar , Indic Scene Text on the Roadside , In Proceeding of International Conference on Document Analysis and Recognition 2024 .
Bibtex
@inproceedings{mondal2024indic,
title={Indic Scene Text on the Roadside},
author={Mondal, Ajoy and Tulsyan, Krishna and Jawahar, CV},
booktitle={International Conference on Document Analysis and Recognition},
pages={263--278},
year={2024},
organization={Springer}
}