Unconstrained Camera Captured Indic Offline Handwritten Dataset
Title: Unconstrained Camera Captured Indic Offline Handwritten Dataset
Authors : Ajoy Mondal and C V Jawahar
Abstract
This paper presents a diverse compilation of Indic offline
handwritten documents. Our dataset comprises 91K handwritten document
images captured through unconstrained camera across thirteen
Indic languages: Assamese, Bengali, Gujarati, Hindi, Kannada, Malayalam,
Manipuri, Marathi, Oriya, Punjabi, Tamil, Telugu, and Urdu, contributed
by 1,220 writers. This dataset encompasses 2600K words and
includes 566,187 unique words featuring diverse content types, such as
alphabetic and numeric. Additionally, we establish a high baseline for the
proposed dataset, facilitating evaluation and benchmarking and explicitly focusing on word recognition tasks. Our findings indicate that our
dataset serves as an effective training source to enhance performance on
respective datasets. We will make the code, dataset, and benchmark results available for further exploration and utilization after acceptance of
the paper1
Keywords: Handwritten text recognition · Indic language · Indic script
· camera captured · unconstrained · word recognition · benchmark.
IIIT-Indic-HW-UC Dataset
We create a larger, more diverse dataset of offline handwritten documents captured by cameras, known as the IIIT-Indic-HW-UC dataset. Compared to the previous version, this dataset features increased language coverage, word count, writer diversity, writing conditions, imaging processes, and ground truth annotation. It includes thirteen major Indic languages: Assamese, Bengali, Gujarati, Hindi, Kannada, Malayalam, Manipuri, Marathi, Oriya, Punjabi, Tamil, Telugu, and Urdu. It consists of 200K word images written by more than 50 writers per language. Writers are required to write corresponding handwritten paragraphs in A4 size white pages, with no constraints on writing style. Handwritten pages are captured using a mobile camera instead of a flatbed scanner. Ground truth annotation is provided at the page level, containing bounding boxes, reading order, textual transcriptions, and the language of all words on the page. We discuss more on it in the following subsections.
Data Collection and Annotation
For each language, there are 7K text paragraphs created from the available text corpus23 covering a wide range of topics. Unique id is associated with a paragraph. Each paragraph contains at most 50 words. Users from any geographical area in India have reading and writing capability for any/all 13 Indic languages to be the authentic writers for the data collection. Any authentic writer can write at least 100 and at most 200 paragraphs in a language selected by the writer. The writer must write one paragraph on one A4 sized page. There is no other constraint on the writing. After writing the paragraph(s), the writer takes a picture of the handwritten page with a mobile camera and shares it with us. There are, on average, 50-100 writers for each language to write 7K text paragraphs. Same paragraphs can be written by multiple writers. With the in- volvement of 1220 writers, we collect a diverse set of 91K handwritten document images corresponding to 91K text paragraphs; each document image is anno- tated at the page level. The annotation includes complete text paragraphs and bounding boxes, reading order, textual transcriptions, and the language of all words on the page. A sample annotated handwritten document image is depicted in Fig 2. Fig. 2(a) illustrates the ground truth bounding boxes, Fig. 2(b) shows textual transcription for complete document image, Fig. 2(c) depicts cropped word level images, and finally Fig. 2(d) reveals how the ground truth information (textual transcriptions, bounding boxes, and sequences of words) is stored in JSON format.
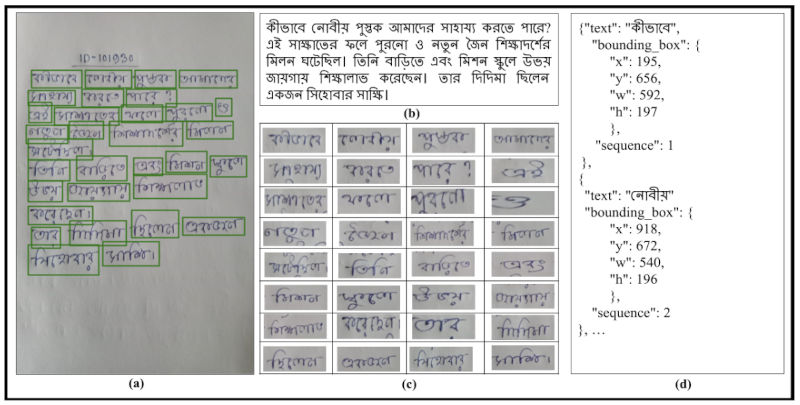
Fig. 2. Illustrates a single Bengali annotated page alongside its standard representa- tion. In (a), a single annotated page from our dataset is depicted. (b) displays the actual text sequence considered as the ground truth. (c) represents all cropped word level images. Lastly, (d) represents textual transcription, bounding box, sequence of words in a page encapsulated within the JSON file.
Feature and Statistics
Since documents are written by various writers all over India, there is enough diversity among documents written by two different writers. Fig. 4 shows a few sample word level images of Hindi written by two different writers: Writer-1 and Writer-21. It highlights that there is still enough variation in writing style and imaging quality between the two writers. Since one writer can write at least 100 and at most 200 pages, for a writer, among document images, there are also enough variations in style and imaging quality because of camera capture. Fig. 5 shows a few sample word level images of Bengali and Hindi languages written by the same writer.
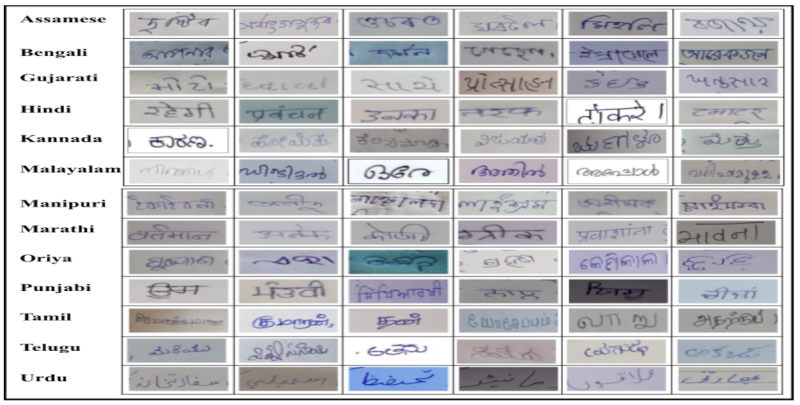
Fig. 3. Show several instances of word level images across various languages, written by multiple writers from our dataset.
Document Image Resolution Distribution: Writers employ their smartphone cameras to photograph handwritten documents, resulting in variations in the resolution of the captured images. Acknowledging that high-resolution document images offer clear text content, facilitate effective model training, and yield superior performance during testing is crucial. Incorporating document images with diverse resolutions ranging from 1600 × 720 to 4608 × 3456 introduces variability in content visibility, thereby enhancing the robustness of the model. Fig. 6(a) highlights the distribution of resolution of handwritten document images by different writers for the Hindi language in our dataset.
Word Level Image Resolution Distribution: Variations in text content and individual writers contribute to differences in the resolution of handwritten word level images. This diversity in word level image resolution improves the model’s generalization ability. As depicted in Fig. 6(b), the distribution of resolutions in Hindi word level images highlights the dataset’s variability. Most word level images have a height-to-width ratio between 0.5 and 1.0, so the dataset encompasses word level images of varying resolutions. Including word level images with diverse resolutions enriches the dataset, enabling the model to accommodate a broader range of visual attributes and enhance its performance across various writing styles and conditions.
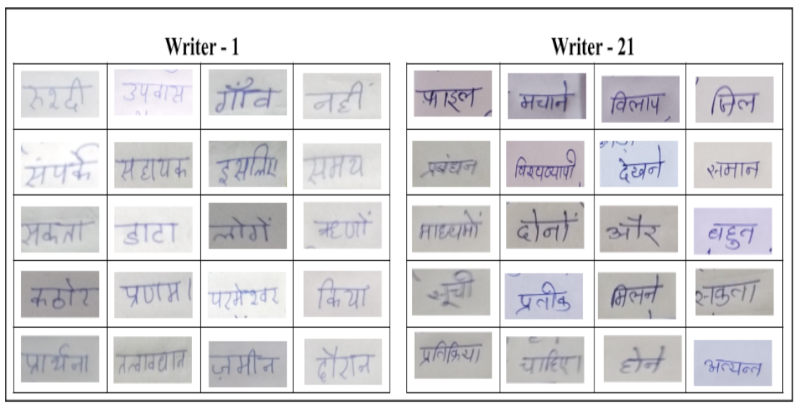
Fig. 4. Presents explicitly sample word level images from just two different writers, namely, writer-1 and writer-21.
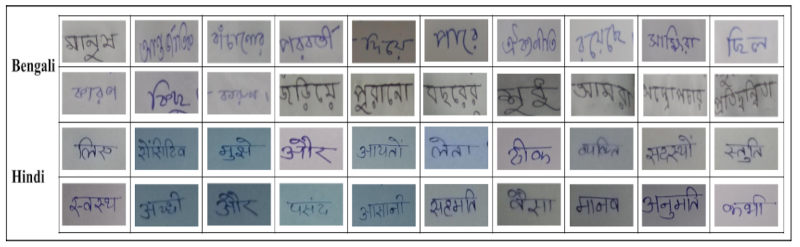
Fig. 5. Show word level images from different document images written by the same writer for Bengali and Hindi languages.
Contrast of Word Level Images: Word level images are extracted from handwritten document images captured by various mobile cameras, resulting in significant variations in intensities and contrast among the images. To evaluate the recognition ease of each word level image, we adopt the global contrast strategy [15]. Fig. 6(c) illustrates the diverse global contrast levels observed among word level images in Hindi. The figures illustrate contrast levels ranging between 10 and 80. This variability in intensity within word level images adds complexity to the dataset, contributing to the development of robust HTR models. However, comprehending and utilizing these variations can enhance the adaptability and effectiveness of HTR algorithms across diverse linguistic contexts.
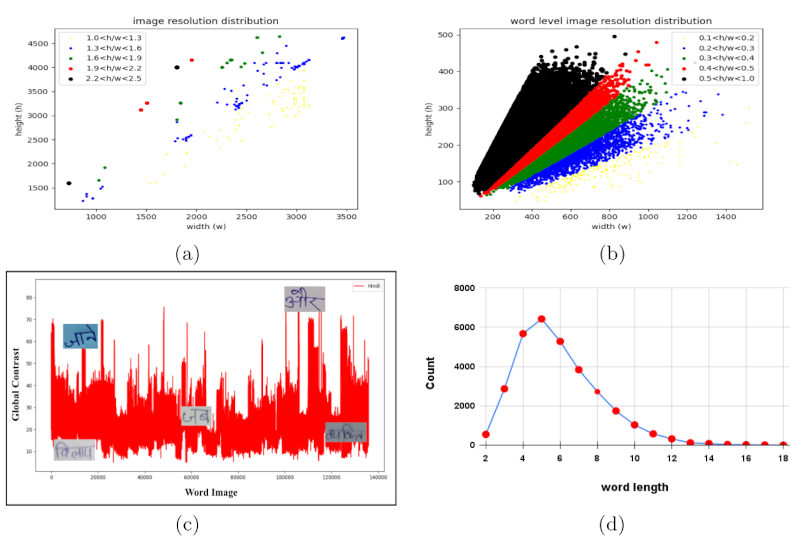
Fig. 6. Shows (a) document image resolution distribution, (b) word level image resolu- tion distribution, (c) varying global contrast among word images, and (d) distribution of word length for the Hindi language in our dataset.
Text Distribution: We compile a dataset of 7K document images for each language, totaling 91K. Additionally, there are 200K word level images per language, resulting in 2600K word level images encompassing unique 566,187 alphabetic and numeric words. Table 1 presents the unique words for each language in our dataset, denoted by the 7th column. For the Hindi language, we include a plot in Fig. 7 that shows the occurrence of unique words in the dataset. The x-axis shows unique words, and the y-axis shows the occurrence of a particular word on a logarithmic scale. This plot demonstrates a long-tail distribution, confirming the diversity of the dataset4 .
Writer Characteristics: Across India, 1,220 individuals have actively con- tributed to curate handwritten document images, resulting in a diverse dataset encompassing various handwriting styles, camera specifications, scanning meth- ods, and more. Among these contributors, 70% (854 individuals) are female, while the remaining 30% (366 individuals) are male. Within the male cohort, 23 individuals are identified as left-handed, with 343 being right-handed. Among the female contributors, 34 individuals are left-handed, while the majority, specifically 820, are right-handed. Notably, a significant portion of the contributors falls within the age range between 20 to 40.
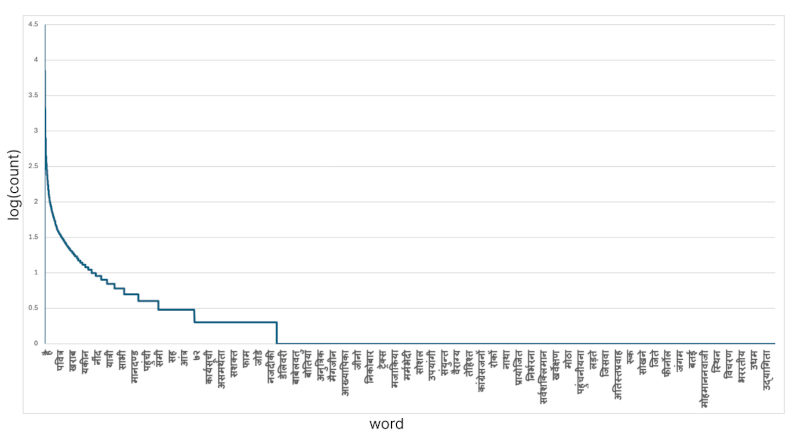
Fig. 7. Shows distribution of unique Hindi words in the dataset.
Dataset Splits: To furnish an extensive training dataset for deep learning models, our dataset, consisting of 2600K word level images, has been partitioned into 1950K word level images for training, 260K word level images for validation, and 390K word level images for testing. For each language, the dataset includes 200K word level images, among them 75% (i.e., 150K), 10% (i.e., 20K), and 15% (i.e., 30K) word images for training, validation, and test sets, respectively.
Comparison with Existing Datasets: Table 1 comprehensively compares our proposed dataset and existing offline Indic handwritten text recognition datasets, highlighting significant disparities and advantages. Various factors, such as dataset size, diversity in handwriting styles, and the inclusion of diverse texts, are meticulously examined. Our IIIT-Indic-HW-UC dataset is twice as large as IIIT-INDIC-HW-WORDS regarding the number of word level im- ages, resulting in a more extensive collection of handwritten document images. In contrast to existing datasets where images are scanned using flatbed scan- ners, our dataset comprises images captured using mobile cameras under un- constrained environments. Camera capture introduces various challenges such as blurred text, overexposed text, perspective distortion, variations in illumination, extensive unwanted backgrounds, low resolution text, text under shadow, and oriented text, among others, in handwritten document images. Across all languages in our dataset, the number of writers exceeds that of IIIT-INDIC- HW-WORDS, indicating a more diverse range of writing styles. For instance, in the Bengali language, the PBOK and CMATERDB2.1 datasets boast more writers (199 and 300, respectively) compared to our dataset, with 158 writers. However, our dataset contains more significant number of unique words (34042) than PBOK and CMATERDB2.1 datasets, which have 925 and 25 unique words, respectively. Similarly, in the Oriya language, while the PBOK dataset has more writers (140) than our dataset (75), our dataset surpasses PBOK in terms of the number of unique words (34074 versus 1040). These distinguishing characteristics render our dataset larger, covering major Indic languages and offering diverse word level images compared to existing datasets.
Results
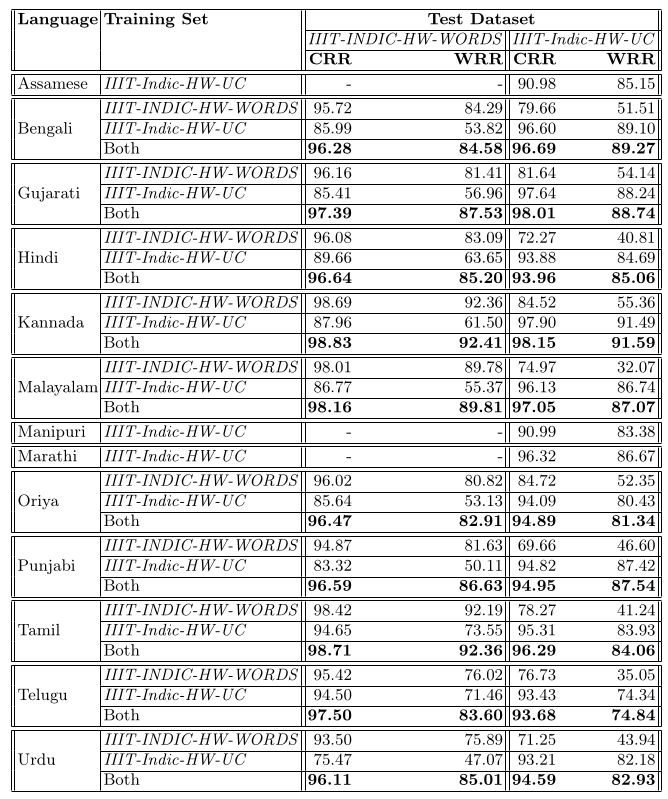
Table 3. Quantitative results on different Indic handwritten datasets. Bold value indicates the best results.
Link to the Download: [ Code ] & [ pdf ]
Datasets:
[ Assamese ] , [ Bengali ] , [ Gujarati ] , [ Hindi ] , [Kannada ] , [ Malayalam ] , [ Manipuri ] , [Marathi ] , [ Oriya ] , [Punjabi ] , [ Tamil ] , [ Telugu ] , [ Urdu ]
Publication
- Ajoy Mondal and C V Jawahar , Unconstrained Camera Captured Indic Offline Handwritten Dataset , In Proceeding of .
Bibtex
Updated Soon.