Quo Vadis, Skeleton Action Recognition ?
Abstract
n this paper, we study current and upcoming frontiers across the landscape of skeleton-based human action recognition.To begin with, we benchmark state-of-the-art models on the NTU-120 dataset and provide multi-layered assessment of the results. Toexamine skeleton action recognition 'in the wild', we introduce Skeletics-152, a curated and 3-D pose-annotated subset of RGB videossourced from Kinetics-700, a large-scale action dataset. The results from benchmarking the top performers of NTU-120 onSkeletics-152 reveal the challenges and domain gap induced by actions 'in the wild'. We extend our study to include out-of-contextactions by introducing Skeleton-Mimetics, a dataset derived from the recently introduced Mimetics dataset. Finally, as a new frontier foraction recognition, we introduce Metaphorics, a dataset with caption-style annotated YouTube videos of the popular social game DumbCharades and interpretative dance performances. Overall, our work characterizes the strengths and limitations of existing approachesand datasets. It also provides an assessment of top-performing approaches across a spectrum of activity settings and via theintroduced datasets, proposes new frontiers for human action recognition.
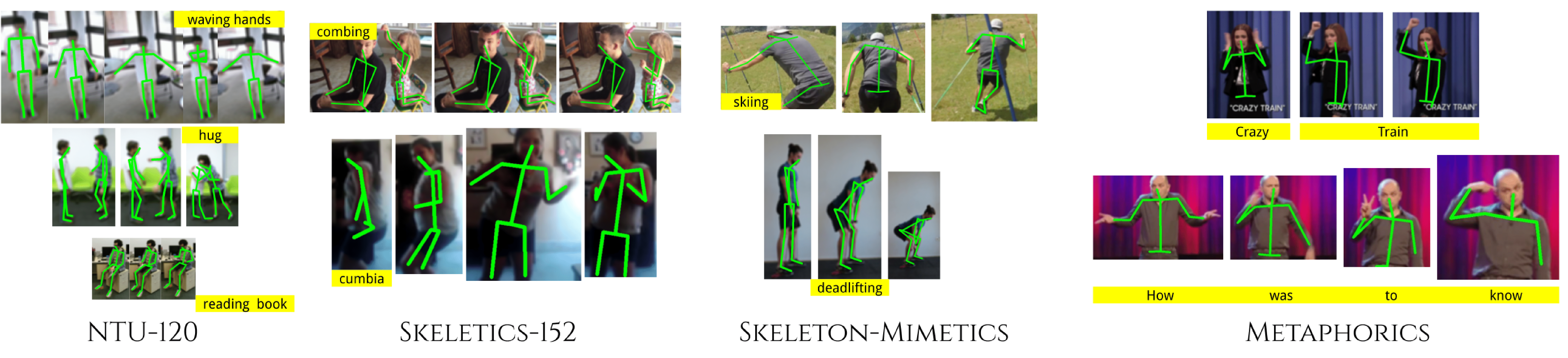
To access the code and paper click here