Spectral Analysis of Brain Graphs
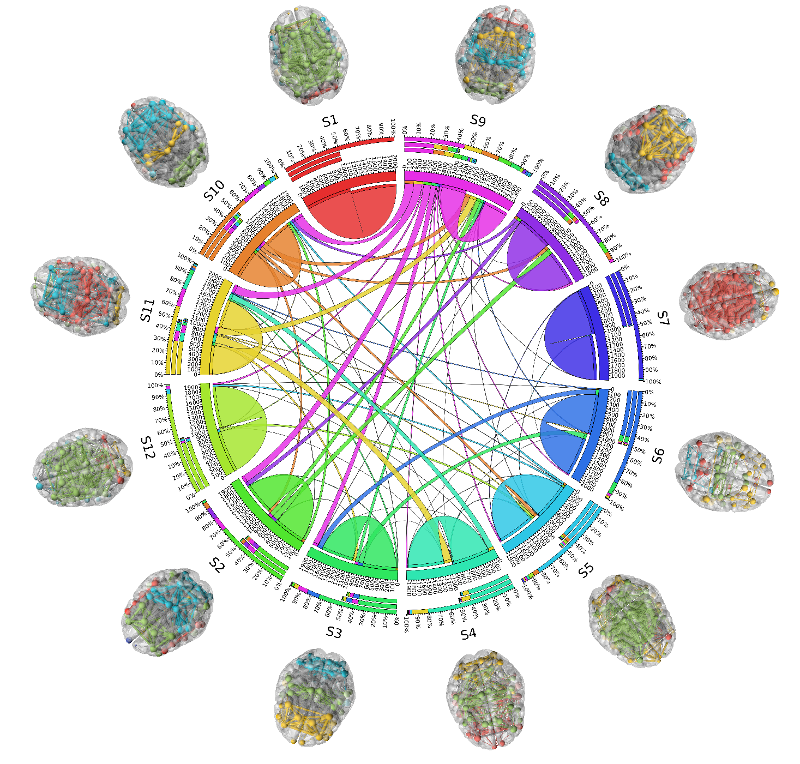
(2) Temporal Multiple Kernel Learning (tMKL) model for predicting resting state FC via characterizing fMRI connectivity dynamics (TMKL)
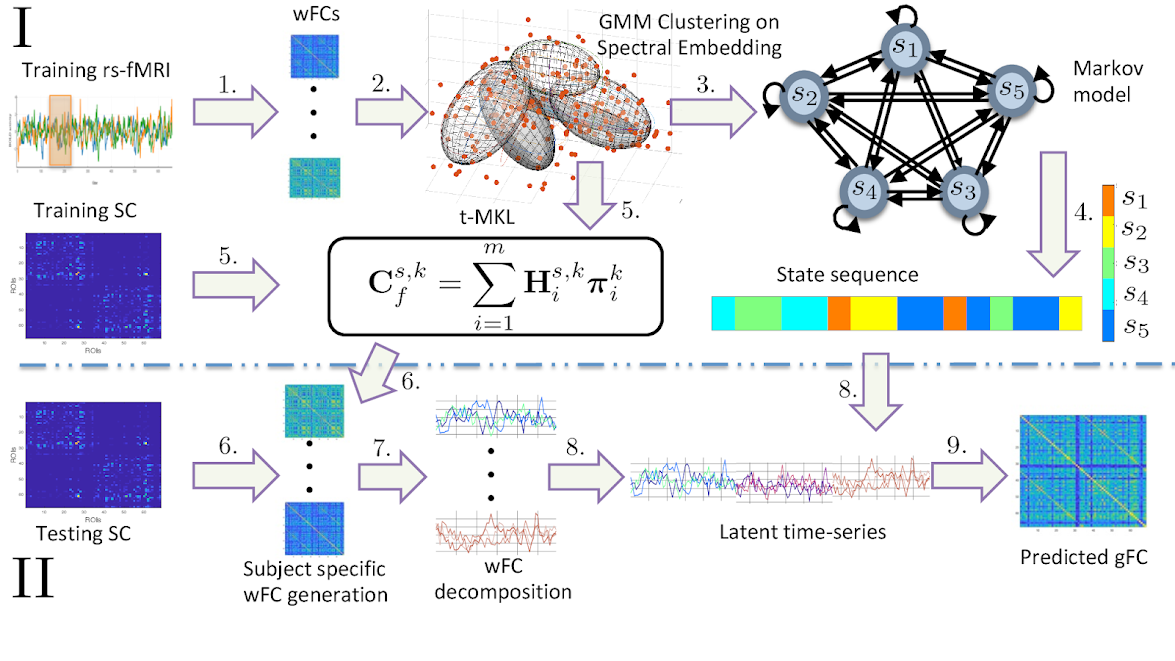
Overview
It has been widely accepted that rs-fMRI signals show non-stationary dynamics over a relatively fixed structural connectome in terms of state transitions. We partition the fMRI timeseries signals using sliding time-windows resulting in time-varying windowed functional connectivity (wFC) matrices. Clusters of all these wFCs are considered as distinct states constituting functional connectivity dynamics (FCD). Transition between states is modeled as a first order Markov process. We use a graph-spectral theoretic approach : temporal - Multiple Kernel Learning model that characterizes FCD as well as predicts the grand average FC or the FC over the entire scanning session of the subject.
Key Findings
- Using Pearson correlation coefficient between empirical and predicted FCs as the measure of model performance, we compared the performance of the proposed model with two existing approaches: our previous project of Multiple Kernel Learning model and the non-linear dynamic-mean-field (DMF) model. Our model performs better than MKL and DMF models (see figure 1).
- Grand average FC predicted by our model also has similar network topology with respect to the empirical FC in terms of corresponding community structures. (see figure 2)
- Community structures in each state show distinct interaction patterns among themselves whose temporal alignment, along with these communities, is recovered by our model, thus playing a major role in the model's superior performance than other models. (see figure 3)
Related Publications
Surampudi, Sriniwas Govinda, Joyneel Misra, Gustavo Deco, Raju Bapi Surampudi, Avinash Sharma, and Dipanjan Roy. "Resting state dynamics meets anatomical structure: Temporal multiple kernel learning (tMKL) model." NeuroImage (2018). Slides Code Paper.
If you use this work, please cite :
@article{surampudi2019resting, title={Resting state dynamics meets anatomical structure: Temporal multiple kernel learning (tMKL) model}, author={Surampudi, Sriniwas Govinda and Misra, Joyneel and Deco, Gustavo and Bapi, Raju Surampudi and Sharma, Avinash and Roy, Dipanjan}, journal={NeuroImage}, volume={184}, pages={609--620}, year={2019}, publisher={Elsevier} }
(1) generalized Multiple Kernel Learning Model (MKL) for Relating SC and FC (MKL)
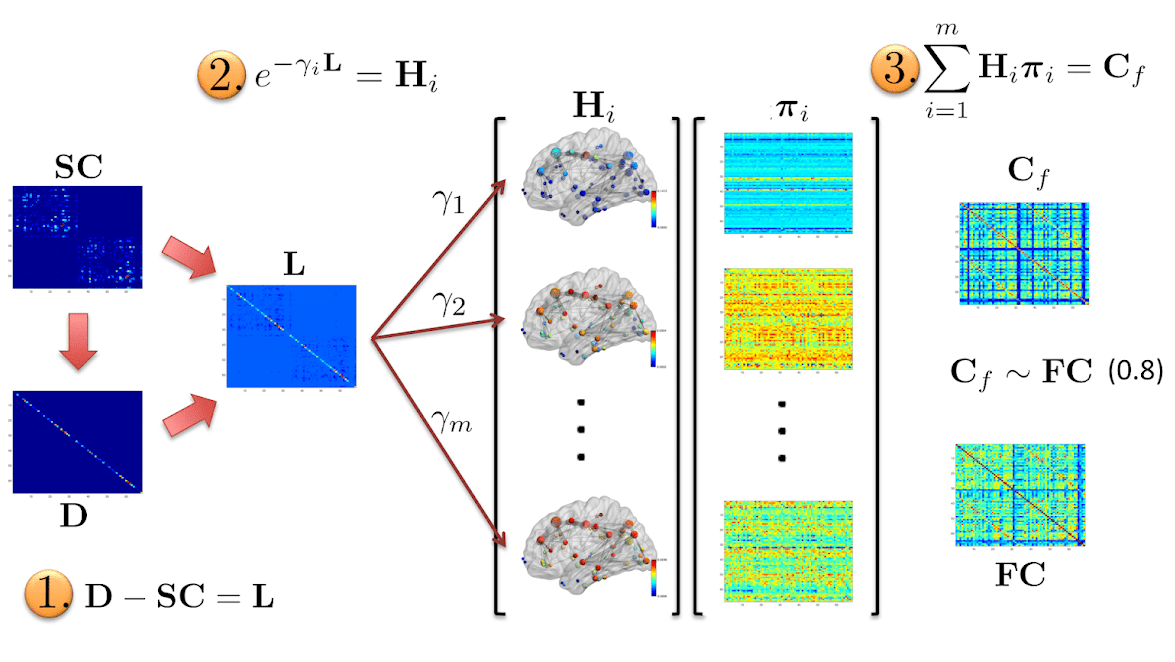
Overview
A challenging problem in cognitive neuroscience is to relate the structural connectivity (SC) to the functional connectivity (FC) to better understand how large-scale network dynamics underlying human cognition emerges from the relatively fixed SC architecture.
We highlight the shortcomings of the existing diffusion models and propose a multi-scale diffusion scheme. Our multi-scale model is formulated as a reaction-diffusion system giving rise to spatio-temporal patterns on a fixed topology. Our model is analytically tractable and complex enough to capture the details of the underlying biological phenomena. We hypothesize the presence of inter-regional co-activations (latent parameters) that combine diffusion kernels at multiple scales to resemble FC from SC.
Key Findings
- We established a bio-physical insight into the spatio-temporal aspect of the graph diffusion kernels.
- Right amount of complexity of the model: Given the strength of the analytical approach and tractability with biological insights, the proposed model could be a suitable method for predicting task-based functional connectivity across different age groups.
- Multi-scale analysis: Latent parameter matrix seems to successfully encapsulate inter- and intra-regional co-activations learned from the training cohort and enables reasonably good prediction of subject-specific empirical functional connectivity (FC) from the corresponding SC matrix.
- Generalized diffusion scheme: Proposed MKL framework encompasses previously proposed diffusion schemes.
Results
- MKL model's FC prediction is better as compared to that of dynamic mean field (DMF) and single diffusion kernel (SDK) (see figure 1a, 1b & 1c).
- Similarity of the FC predicted by the MKL model and the empirical FC in terms of community assignment (see figures 2 & 3).
- Model structure supports rich-club organization (see figure 4).
- Model passes various perturbation tests suggesting its robustness (see figures 5 & 6).
Related Publications
Sriniwas Govinda Surampudi, Shruti Naik, Raju Bapi Surampudi, Viktor K. Jirsa, Avinash Sharma and Dipanjan Roy. "Multiple Kernel Learning Model for Relating Structural and Functional Connectivity in the Brain." Scientific Reports. doi:10.1038/s41598-018-21456-0 Slide Code Paper
If you use this work, please cite :
@article{surampudi2018multiple, title={Multiple Kernel Learning Model for Relating Structural and Functional Connectivity in the Brain}, author={Surampudi, Sriniwas Govinda and Naik, Shruti and Surampudi, Raju Bapi and Jirsa, Viktor K and Sharma, Avinash and Roy, Dipanjan}, journal={Scientific reports}, volume={8}, number={1}, pages={3265}, year={2018}, publisher={Nature Publishing Group} }
Collaborators
Dipanjan Roy
Cognitive Brain Dynamics Lab, NBRC
Personal Page: https://dipanjanr.com/
Openings