IndicSpeech: Text-to-Speech Corpus for Indian Languages
[Dataset]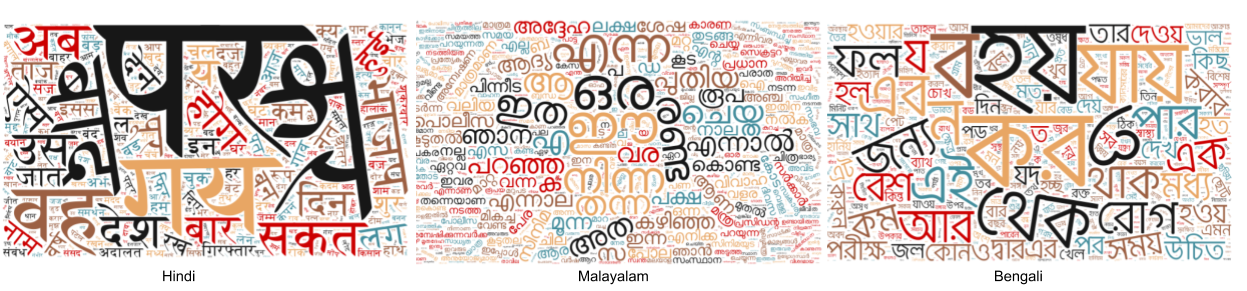
Word clouds of the collected corpus for 3 languages
Abstract
India is a country where several tens of languages are spoken by over a billion strong population. Text-to-speech systems for such languages will thus be extremely beneficial for wide-spread content creation and accessibility. Despite this, the current TTS systems for even the most popular Indian languages fall short of the contemporary state-of-the-art systems for English, Chinese, etc. We believe that one of the major reasons for this is the lack of large, publicly available text-to-speech corpora in these languages that are suitable for training neural text-to-speech systems. To mitigate this, we release a large text-to-speech corpus for $3$ major Indian languages namely Hindi, Malayalam and Bengali. In this work, we also train a state-of-the-art TTS system for each of these languages and report their performances.
Paper
-
IndicSpeech: Text-to-Speech Corpus for Indian Languages
Nimisha Srivastava, Rudrabha Mukhopadhyay*, Prajwal K R*, C.V. Jawahar
IndicSpeech: Text-to-Speech Corpus for Indian Languages, LREC, 2020
[PDF] | [BibTeX]@inproceedings{srivastava-etal-2020-indicspeech,
title = "{I}ndic{S}peech: Text-to-Speech Corpus for {I}ndian Languages",
author = "Srivastava, Nimisha and
Mukhopadhyay, Rudrabha and
K R, Prajwal and
Jawahar, C V",
booktitle = "Proceedings of The 12th Language Resources and Evaluation Conference",
month = may,
year = "2020",
address = "Marseille, France",
publisher = "European Language Resources Association",
url = "https://www.aclweb.org/anthology/2020.lrec-1.789",
pages = "6417--6422",
abstract = "India is a country where several tens of languages are spoken by over a billion strong population. Text-to-speech systems for such languages will thus be extremely beneficial for wide-spread content creation and accessibility. Despite this, the current TTS systems for even the most popular Indian languages fall short of the contemporary state-of-the-art systems for English, Chinese, etc. We believe that one of the major reasons for this is the lack of large, publicly available text-to-speech corpora in these languages that are suitable for training neural text-to-speech systems. To mitigate this, we release a 24 hour text-to-speech corpus for 3 major Indian languages namely Hindi, Malayalam and Bengali. In this work, we also train a state-of-the-art TTS system for each of these languages and report their performances. The collected corpus, code, and trained models are made publicly available.",
language = "English",
ISBN = "979-10-95546-34-4",
}
Live Demo
Please click here for demo video : https://bhaasha.iiit.ac.in/indic-tts/
Contact
- Prajwal K R -
This email address is being protected from spambots. You need JavaScript enabled to view it. - Rudrabha Mukhopadhyay -
This email address is being protected from spambots. You need JavaScript enabled to view it.