CVPR 2016 Paper - First Person Action Recognition Using Deep Learned Descriptors
Abstract
We focus on the problem of wearer’s action recognition in first person a.k.a. egocentric videos. This problem is more challenging than third person activity recognition due to unavailability of wearer’s pose, and sharp movements in the videos caused by the natural head motion of the wearer. Carefully crafted features based on hands and objects cues for the problem have been shown to be successful for limited targeted datasets. We propose convolutional neural networks (CNNs) for end to end learning and classification of wearer’s actions. The proposed network makes use of egocentric cues by capturing hand pose, head motion and saliency map. It is compact. It can also be trained from relatively small labeled egocentric videos that are available. We show that the proposed network can generalize and give state of the art performance on various disparate egocentric action datasets.
Method
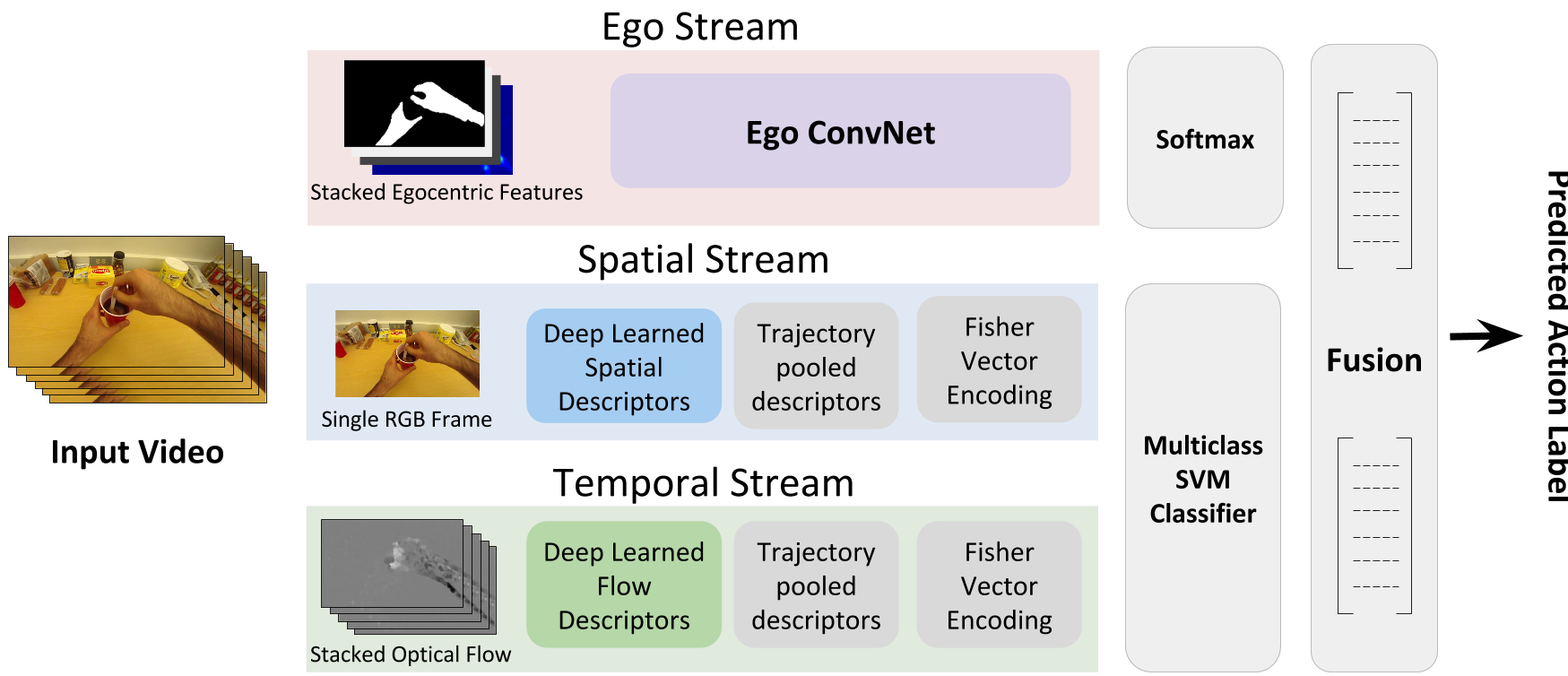
Paper
Downloads
Code
Datasets and annotations
- GTEA
-
Kitchen [Labels] *
-
ADL *
- P_04 [Annotation]
- P_05 [Annotation]
- P_06 [Annotation]
- P_09 [Annotation]
- P_11 [Annotation]
-
UTE *
- P01 [Annotation]
- P03 [Annotation]
- P032 [Annotation]
PR 2016 Paper - Trajectory Aligned Features For First Person Action Recognition
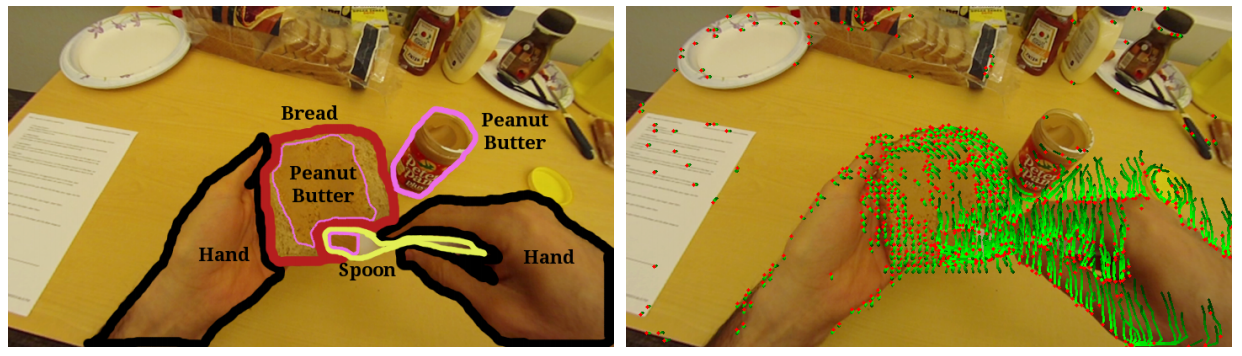
Abstract
Egocentric videos are characterised by their ability to have the first person view. With the popularity of Google Glass and GoPro, use of egocentric videos is on the rise. Recognizing action of the wearer from egocentric videos is an important problem. Unstructured movement of the camera due to natural head motion of the wearer causes sharp changes in the visual field of the egocentric camera causing many standard third person action recognition techniques to perform poorly on such videos. Objects present in the scene and hand gestures of the wearer are the most important cues for first person action recognition but are difficult to segment and recognize in an egocentric video. We propose a novel representation of the first person actions derived from feature trajectories. The features are simple to compute using standard point tracking and does not assume segmentation of hand/objects or recognizing object or hand pose unlike in many previous approaches. We train a bag of words classifier with the proposed features and report a performance improvement of more than 11% on publicly available datasets. Although not designed for the particular case, we show that our technique can also recognize wearer's actions when hands or objects are not visible.
Paper
Downloads
Code
Dataset and Annotations
NCVPRIPG 2015 Paper - Generic Action Recognition from Egocentric Videos
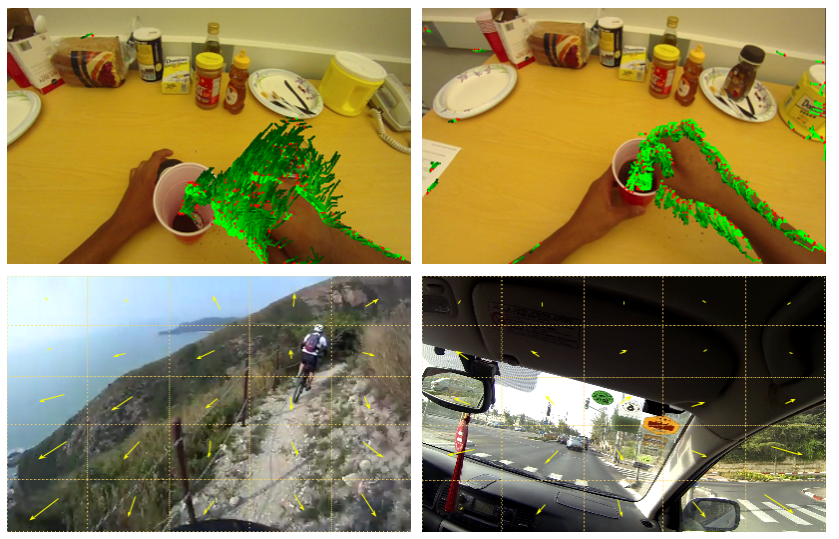
Abstract
Egocentric cameras are wearable cameras mounted on a person’s head or shoulder. With their ability to have first person view, such cameras are spawning new set of exciting applications in computer vision. Recognising activity of the wearer from an egocentric video is an important but challenging problem. The task is made especially difficult because of unavailability of wearer’s pose as well as extreme camera shake due to motion of wearer’s head. Solutions suggested so far for the problem, have either focussed on short term actions such as pour, stir etc. or long term activities such as walking, driving etc. The features used in both the styles are very different and the technique developed for one style often fail miserably on other kind. In this paper we propose a technique to identify if a long term or a short term action is present in an egocentric video segment. This allows us to have a generic first-person action recognition system where we can recognise both short term as well as long term actions of the wearer. We report an accuracy of 90.15% for our classifier on publicly available egocentric video dataset comprising 18 hours of video amounting to 1.9 million tested samples.