![]() |
![]() |
![]() |
Abstract
The notion of relative attributes as introduced by Parikh and Grauman (ICCV, 2011) provides an appealing way of comparing two images based on their visual properties (or attributes) such as “smiling” for face images, “naturalness” for outdoor images, etc. For learning such attributes, a Ranking SVM based formulation was proposed that uses globally represented pairs of annotated images. In this paper, we extend this idea towards learning relative attributes using local parts that are shared across categories.First, instead of using a global representation, we introduce a part-based representation combining a pair of images that specifically compares corresponding parts. Then, with each part we associate a locally adaptive “significance coefficient” that represents its discriminative ability with respect to a particular attribute. For each attribute, the significance-coefficients are learned simultaneously with a max-margin ranking model in an iterative manner. Compared to the baseline method , the new method is shown to achieve significant improvements in relative attribute prediction accuracy. Additionally, it is also shown to improve relative feedback based interactive image search.
CONTRIBUTIONS
- Extend the idea of relative attributes (Parikh and Grauman ) to localized parts.
- For each part we associate a locally adaptive “significance coefficient” that are learned simultaneously with a max-margin ranking model in an iterative manner.
- Our method gives significant improvement (more than 10% on absolute scale) compared to the baseline method.
- Introduce a new LFW-10 data set that has 10,000 pairs with instance-level annotations for 10 attributes.
- Demonstrate application to interactive image search.
Method
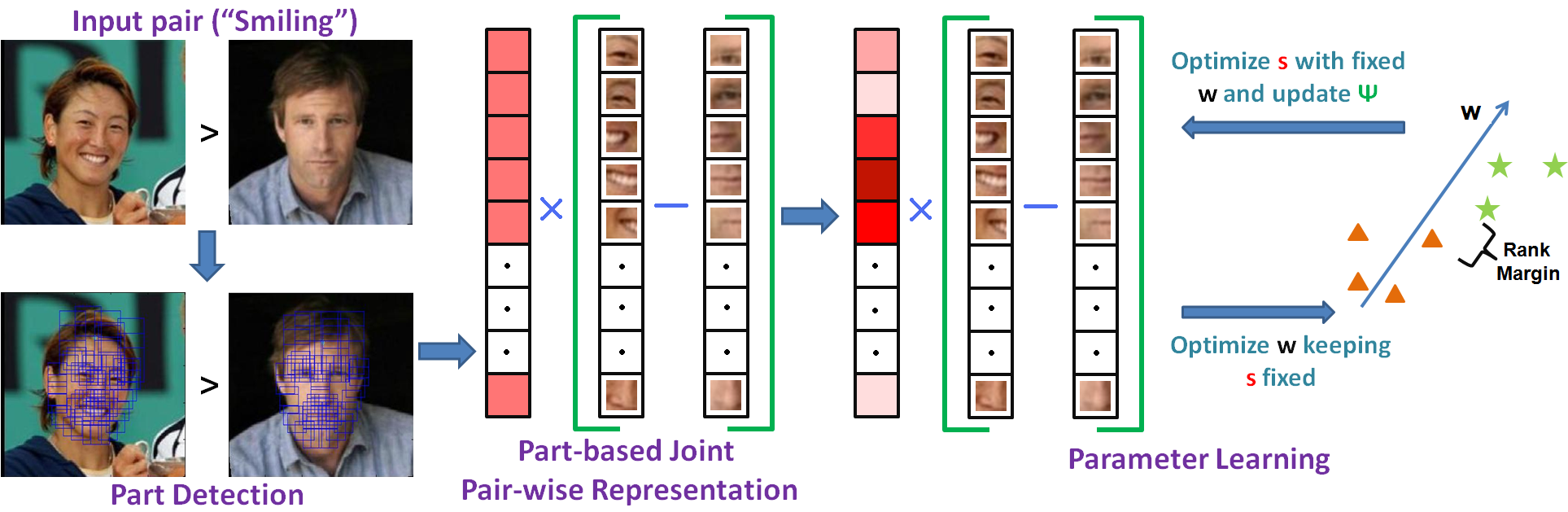
Dataset
We randomly select 2000 images from LFW dataset . Out of these, 1000 images are used for creating training pairs and the remaining (unseen) 1000 for testing pairs. The annotations are collected for 10 attributes, with 500 training and testing pairs per attribute. In order to minimize the chances of inconsistency in the dataset, each image pair is got annotated from 5 trained annotators, and final annotation is decided based on majority voting.
Relative Parts: Distinctive Parts for Learning Relative Attributes (CVPR, 2014)
Publication
Relative Parts: Distinctive Parts for Learning Relative Attributes
IEEE Conference on Computer Vision and Pattern Recognition (CVPR), 2014
Results

Top 10 parts learned using our method with maximum weights for each of the ten attributes in LFW-10 dataset. Greater is the intensity of red, more important is that part, and vice-versa.
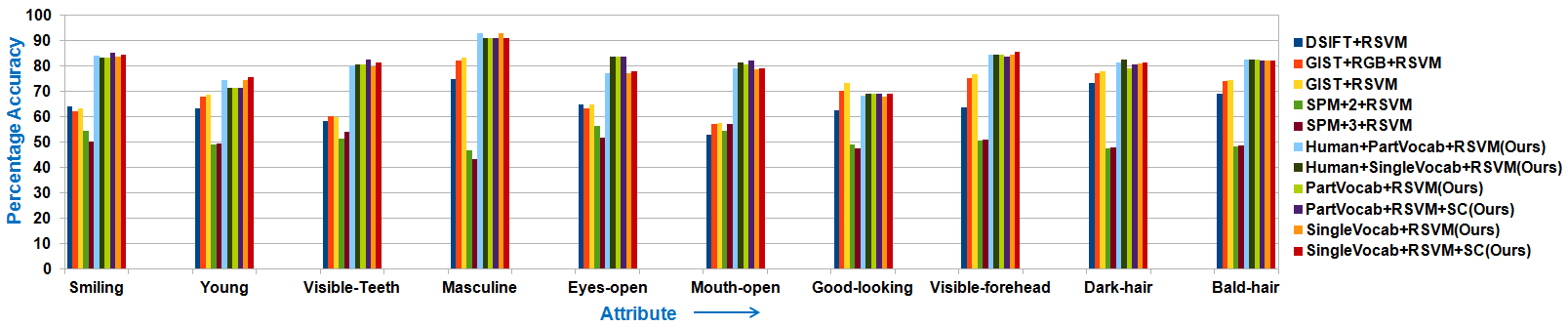
Performance for each of the ten attributes in LFW-10 dataset using different methods and representations.
People
- Sandeep
- Yashaswi Verma
- C. V. Jawahar
Acknowledgement
Yashaswi Verma is partly supported by MSR India PhD Fellowship 2013.
Copyright Notice
The documents contained in these directories are included by the contributing authors as a means to ensure timely dissemination of scholarly and technical work on a non-commercial basis. Copyright and all rights therein are maintained by the authors or by other copyright holders, notwithstanding that they have offered their works here electronically. It is understood that all persons copying this information will adhere to the terms and constraints invoked by each author's copyright.