N2NSkip: Learning Highly Sparse Networks using Neuron-to-Neuron Skip Connections
Owing to the overparametrized nature and high memory requirements of classical DNNs, there has been a renewed interest in network sparsification. Is it possible to prune a network at initialization (prior to training) while maintaining rich connectivity, and also ensure faster convergence? We attempt to answer this question by emulating the pattern of neural connections in the brain.
Figure: After a preliminary pruning step, N2NSkip connections are added to the pruned network while maintaining the overall sparsity of the network.
Abstract
The over-parametrized nature of Deep Neural Networks (DNNs) leads to considerable hindrances during deployment on low-end devices with time and space constraints. Network pruning strategies that sparsify DNNs using iterative prune-train schemes are often computationally expensive. As a result, techniques that prune at initialization, prior to training, have become increasingly popular. In this work, we propose neuron-to-neuron skip (N2NSkip) connections, which act as sparse weighted skip connections, to enhance the overall connectivity of pruned DNNs. Following a preliminary pruning step, N2NSkip connections are randomly added between individual neurons/channels of the pruned network, while maintaining the overall sparsity of the network. We demonstrate that introducing N2NSkip connections in pruned networks enables significantly superior performance, especially at high sparsity levels, as compared to pruned networks without N2NSkip connections. Additionally, we present a heat diffusion-based connectivity analysis to quantitatively determine the connectivity of the pruned network with respect to the reference network. We evaluate the efficacy of our approach on two different preliminary pruning methods which prune at initialization, and consistently obtain superior performance by exploiting the enhanced connectivity resulting from N2NSkip connections.
Methods:
In this work, inspired by the pattern of skip connections in the brain, we propose sparse, learnable neuron-to-neuron skip (N2NSkip) connections, which enable faster convergence and superior effective connectivity by improving the overall gradient flow in the pruned network. N2NSkip connections regulate overall gradient flow by learning the relative importance of each gradient signal, which is propagated across non-consecutive layers, thereby enabling efficient training of networks pruned at initialization (prior to training). This is in contrast with conventional skip connections, where gradient signals are merely propagated to previous layers. We explore the robustness and generalizability of N2NSkip connections to different preliminary pruning methods and consistently achieve superior test accuracy and higher overall connectivity. Additionally, our work also explores the concept of connectivity in deep neural networks through the lens of heat diffusion in undirected acyclic graphs. We propose to quantitatively measure and compare the relative connectivity of pruned networks with respect to the reference network by computing the Frobenius norm of their heat diffusion signatures at saturation.
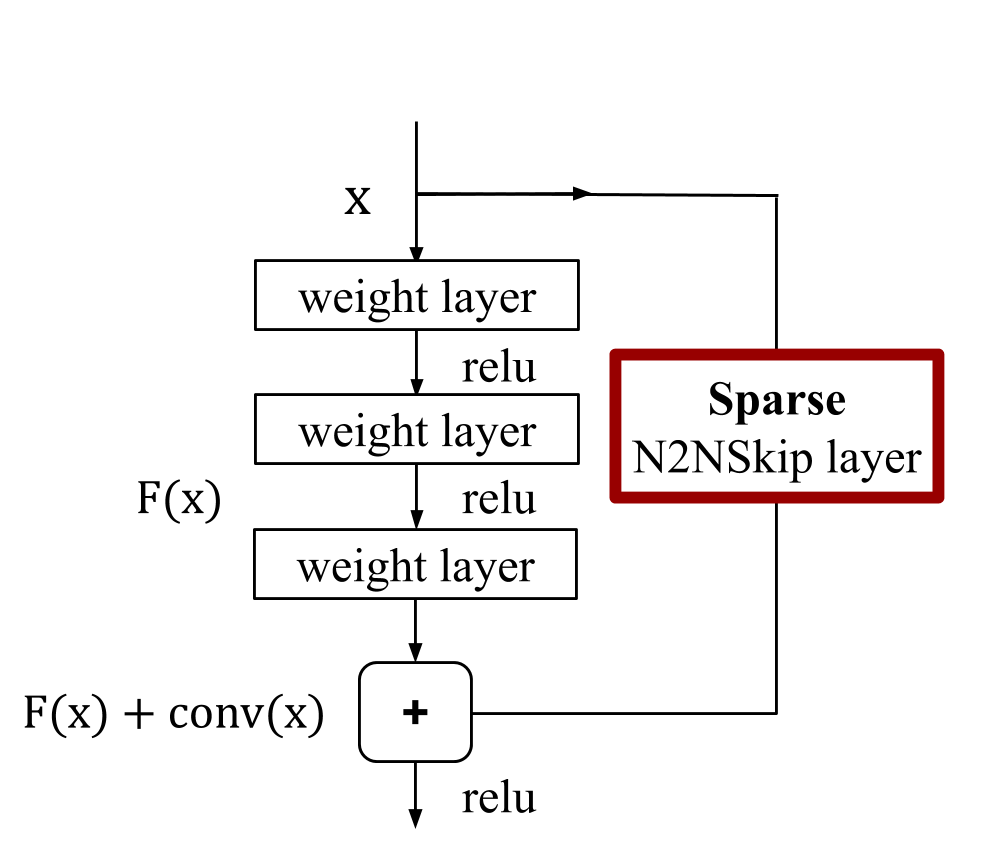
Figure: As opposed to conventional skip connections, N2NSkip connections introduce skip connections between non-consecutive layers of the network, and are parametrized by sparse learnable weights.
Contributions:
- We propose N2NSkip connections which significantly improve the effective connectivity and test performance of sparse networks across different datasets and network architectures. Notably, we demonstrate the generalizability of N2NSkip connections to different preliminary pruning methods and consistently obtain superior test performance and enhanced overall connectivity.
- We propose a heat diffusion-based connectivity measure to compare the overall connectivity of pruned networks with respect to the reference network. To the best of our knowledge, this is the first attempt at modeling connectivity in DNNs through the principle of heat diffusion.
- We empirically demonstrate that N2NSkip connections significantly lower performance degradation as compared to conventional skip connections, resulting in consistently superior test performance at high compression ratios
Visualizing Adjacency Matrices
Considering each network as an undirected graph, we construct an n × n adjacency matrix, where n is the total number of neurons in the MLP. To verify the enhanced connectivity resulting from N2NSkip connections, we compare the heat diffusion signature of the pruned adjacency matrices with the heat diffusion signature of the reference network.
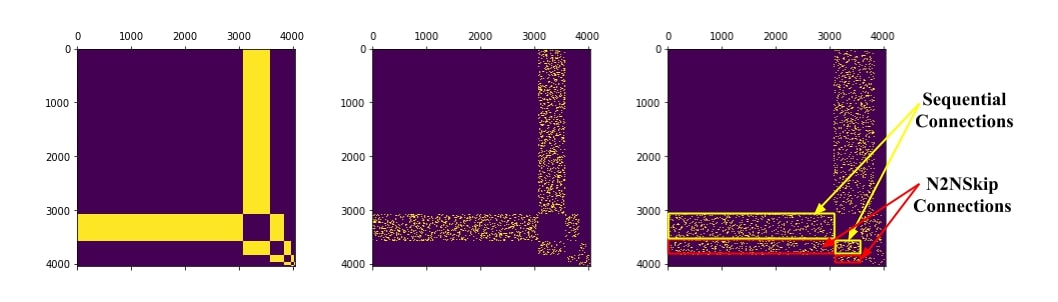
Figure: Binary adjacency matrices for (a) Reference network (MLP) (b) Pruned network at a compression of 5x (randomized pruning) (c) N2NSkip network at a compression of 5x (10% N2NSkip connections + 10% sequential connections).
Experimental Results:
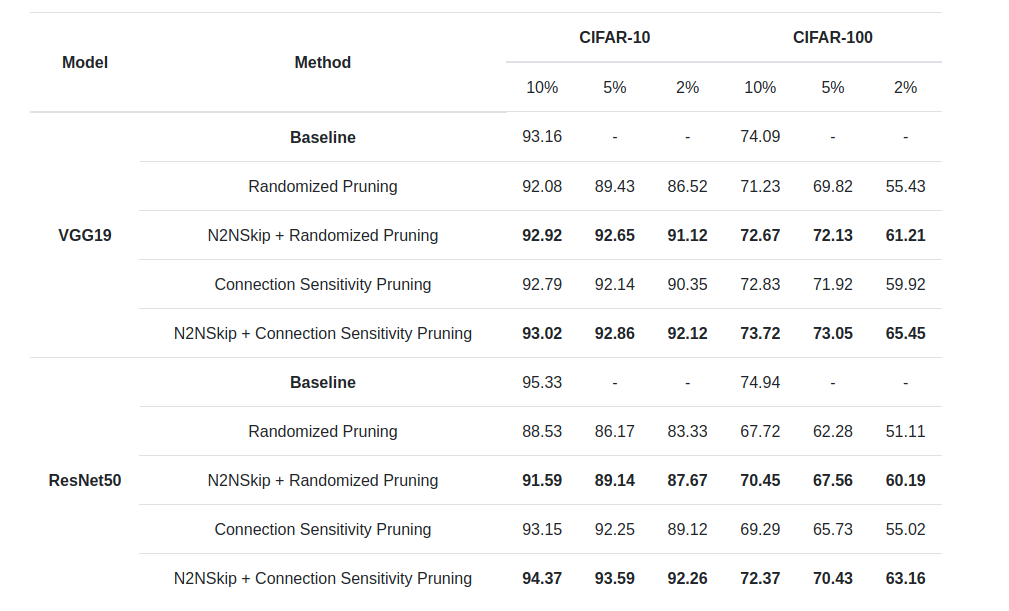
Test Accuracy of pruned ResNet50 and VGG19 on CIFAR-10 and CIFAR-100 with either RP or CSP as the preliminary pruning step. The addition of N2NSkip connections leads to a significant increase in test accuracy. Additionally, there is a larger increase in accuracy at network densities of 5% and 2%, as compared to 10%. This observation is consistent for both N2NSkip-RP and N2NSkip-CSP, which indicates that N2NSkip connections can be used as a powerful tool to enhance the performance of pruned networks at high compression rates.
Related Publication:
- Arvind Subramaniam, Avinash Sharma - N2NSkip: Learning Highly Sparse Networks using Neuron-to-Neuron Skip Connections, British Machine Vision Conference (BMVC 2020).