Handwriting Analysis
Introduction
The work in handwriting analysis at CVIT concentrates on Recognition, Synthesis, Annotation, Search, and Classification of handwritten data. We primarily concentrate on online handwriting, where the temporal information of the writing process is available in the handwritten data, although many of the approaches we use are extensible to offline handwriting as well. Specifically, recognition of online handwriting in Indian languages has special significance, as it can form an effective mechanism of data input, as opposed to keyboards that needs multiple keystrokes and control sequences to input many characters.
Handwriting Synthesis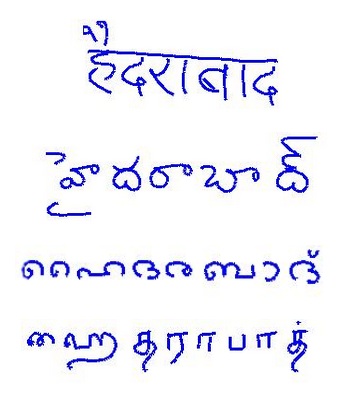
Handwriting synthesis is the problem of generating data close to how a human would write the text. The characterstics of the generated data could be that of specific writer or that from a generic model. Systhesis of handwriting pose a challange as writer spcecific features need to captured and preserved, yet at the same time, variability between handwriting should also be taken into account. Even with the given model, synthesis should not be deterministic since the variation that are found in human handwriting are stochastic.
Application of handwriting synthesis, includes, automatically creation of personalized handwritten documents, large amount of annotated handwritten data for training of recognition engines and writer independent matching and retrieval of handwritten documents.
For synthesis of Indic scripts, we model the handwriting at two levels. A stroke level model is used to capture the writing style and hand movements of the individual strokes. A space-time layout model is then used to arrange the synthesized strokes to form the words. Both the stroke model and the layout model can be learned from examples, and the method can learn from a single example, as well as a large collection to capture the variations. The model also allows us to synthesize the words in multiple Indic scripts through transliteration.
Annotation and Search of Handwritten data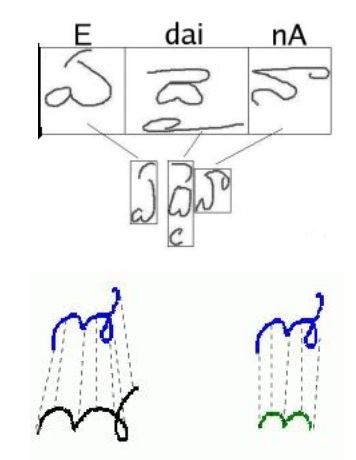
Annotation of handwriting is the process of labeling input data for training for the purpose of a variety of handwriting analysis problems like Handwriting recognition and writer identification systems. However manual annotation of large datasets is tedious, expensive, and error prone process, especially at character and stroke level. Lack of proper linguistic resources in form of annotated data sets is a major hurdle in building recognizers for them.
In many practical situations, plain transcripts of handwritten data is available, which can be used to make the process of annotation, easier. Data collection process can be carried out in different setting like, unrestricted data, designed text, dictation, and data generation (using handwriting synthesis). A parallel text is available in all the above case, except that of unrestricted data. For annotation, we use the model based handwriting synthesis unit described above to map the text corpora to handwriting space and annotation is propagated to word and character levels using elastic matching of handwriting. Stroke level annotation for online handwriting recognition is currently being done using semi-automatic tools.
Online Handwriting Recognition
We aim at building robust and accurate recognition engine for Indian languages, specifically for Hindi, Telugu and Malayalam. There are some features for Indian Languages and their writing, which necessitates a different approach for recognition as compared to English:
- The primary unit of words is an akshara, which is a combination of multiple consonants, and ending in a vowel.
- Each language has a very large set of aksharas - usually multiple thousands
- Each akshara is composed of a single or multiple strokes, and no partial strokes.
A robust and accurate recognition system poses a variety of research challenges, especially for Indian languages. We concentrate on a variety of problems such as building large-class hierarchical classifiers, specifically for handwriting recognition and OCR, Discriminating classifiers for differenting similar looking time-series data (strokes), Compact representation of class models, Efficient spell checkers for languages with large number of work form variations, etc.
Writer Identification
Writer Identification is a process of identifying the authorship of handwritten documents. Relevance of document in civil and criminal litigations, primarily dependent on our ability to assign authorship to the particular document. For more information on writer identification click here.
Related Publications
Anoop M. Namboodiri and Sachin Gupta - Text Independent Writer Identification from Online Handwriting, International Workshop on Frontiers in Handwriting Recognition(IWFHR'06), October 23-26, 2006, La Baule, Centre de Congreee Atlantia, France. [PDF]
Anand Kumar, A. Balasubramanian, Anoop M. Namboodiri and C.V. Jawahar - Model-Based Annotation of Online Handwritten Datasets, International Workshop on Frontiers in Handwriting Recognition(IWFHR'06), October 23-26, 2006, La Baule, Centre de Congreee Atlantia, France. [PDF]
Karteek Alahari, Satya Lahari Putrevu and C.V. Jawahar - Learning Mixtures of Offline and Online Features for Handwritten Stroke Recognition, Proc. 18th IEEE International Conference on Pattern Recognition(ICPR'06), Hong Kong, Aug 2006, Vol. III, pp.379-382. [PDF]
C. V. Jawahar and A. Balasubramanian - Synthesis of Online Handwriting in Indian Languages, International Workshop on Frontiers in Handwriting Recognition(IWFHR'06), October 23-26, 2006, La Baule, Centre de Congree Atlantia, France. [PDF]
Karteek Alahari, Satya Lahari P and C. V. Jawahar - Discriminant Substrokes for Online Handwriting Recognition, Proceedings of Eighth International Conference on Document Analysis and Recognition(ICDAR), Seoul, Korea 2005, Vol 1, pp 499-503. [PDF]
A. Bhaskarbhatla, S. Madhavanath, M. Pavan Kumar, A. Balasubramanian, and C. V. Jawahar - Representation and Annotation of Online Handwritten Data, Proceedings of the International Workshop on Frontiers in Handwriting Recognition(IWFHR), Oct. 2004, Tokyo, Japan, pp. 136--141. [PDF]
Pranav Reddy and C. V. Jawahar, The Role of Online and Offline Features in the Development of a Handwritten Signature Verification System, Proceedings of the National Conference on Document Analysis and Recognition(NCDAR), Jul. 2001, Mandya, India, pp. 85--94. [PDF]
Associated People
|
|
|