Word level Handwritten datasets for Indic scripts
Abstract
Handwriting recognition (HWR) in Indic scripts is a challenging problem due to the inherent subtleties in the scripts, cursive nature of the handwriting and similar shape of the characters. Lack of publicly available handwriting datasets in Indic scripts has affected the development of handwritten word recognizers. In order to help resolve this problem, we release 2 handwritten word datasets: IIIT-HW-Dev, a Devanagari dataset and IIIT-HW-Telugu, a Telugu dataset.
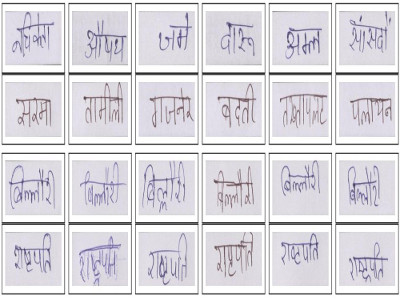
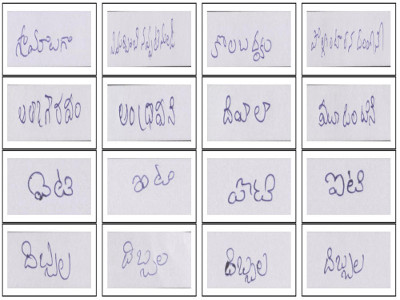
Major Contributions
- A Devanagari dataset comprising of over 95K handwritten words.
- A Telugu dataset comprising comprising of over 120K handwritten words.
- The dataset is benchmarked using existing state-of-the art methods for HWR Recognition
Related Publications
- Kartik Dutta, Praveen Krishnan, Minesh Mathew and CV Jawahar, Offline Handwriting Recognition on Devanagari using a new Benchmark Dataset, International Workshop on Document Analysis Systems (DAS) 2018 .
- Kartik Dutta, Praveen Krishnan, Minesh Mathew and C.V Jawahar, Towards Spotting and Recognition of Handwritten Words in Indic Scripts , International Conference on Frontiers of Handwriting Recognition ( ICFHR) 2018
Dataset:
Each dataset contains a Readme file that explains the different files in the dataset. Both the datasets contain word images only and these images are in jpg format.
Version 1
- Devanagiri Dataset (IIIT-HW-Dev) [Dataset] (File size: 1.8 GB)
- Telugu Dataset (IIIT-HW-Telugu) [Dataset] (File size: 3.7 GB)
Changes in Version 1
Corrected segmentation errors occurring in the datasets.
Version 0
Bibtex
If you use this work or dataset, please cite :
@inproceedings{IIITHWDev2018, title={ Offline Handwriting Recognition on Devanagari using a new Benchmark Dataset }, author={Dutta, Kartik and Krishnan, Praveen and Mathew, Minesh and Jawahar, C.~V.}, booktitle={DAS}, year={2018} } @inproceedings{IIITHWTelugu2018, title={ Towards Spotting and Recognition of Handwritten Words in Indic Scripts }, author={Dutta, Kartik and Krishnan, Praveen and Mathew, Minesh and Jawahar, C.~V.}, booktitle={ICFHR}, year={2018} }