Towards Structured Analysis of Broadcast Badminton Videos
Abstract
We propose a method to analyze a large corpus of badminton broadcast videos by segmenting the points played, tracking and recognizing the players in each point and annotating
their respective badminton strokes. We evaluate the performance on 10 Olympic matches with 20 players and achieved 95.44% point segmentation accuracy, 97.38% player detection score (mAP@0.5), 97.98% player identification accuracy, and stroke segmentation edit scores of 80.48%. We further show that the automatically annotated videos alone could enable the gameplay analysis and inference by computing understandable metrics such as player’s reaction time, speed, and footwork around the court, etc.
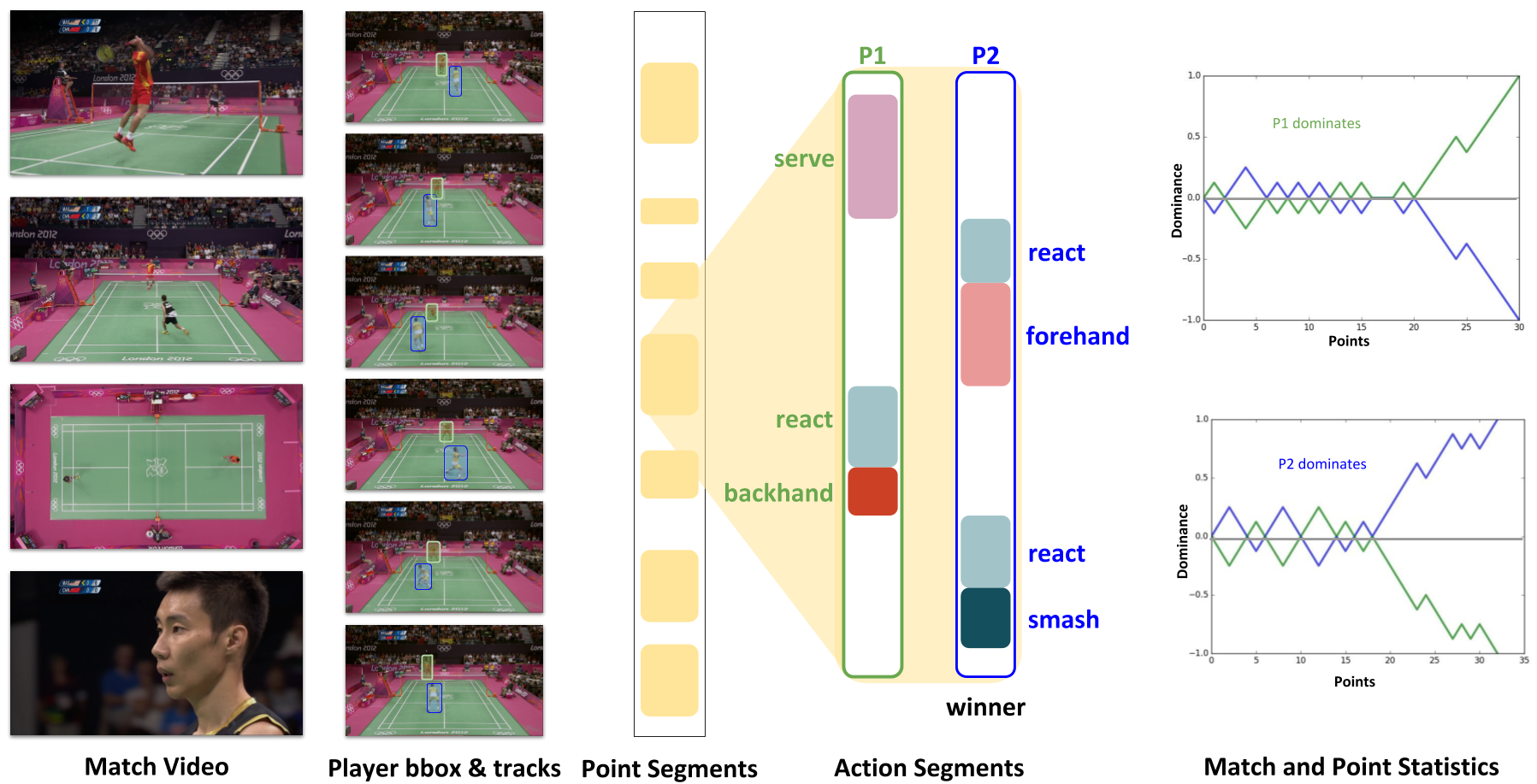
Related Publications
- Anurag Ghosh, Suriya Singh and C.V. Jawahar, Towards Structured Analysis of Broadcast Badminton Videos, IEEE Winter Conference on Applications of Computer Vision (WACV 2018), Lake Tahoe, CA, USA, 2018. [PDF] [Supp]
Bibtex
If you use this work or dataset, please cite :
@inproceedings{ghosh2018towards, title={Towards Structured Analysis of Broadcast Badminton Videos}, author={Ghosh, Anurag and Singh, Suriya and Jawahar, C.~V.}, booktitle={IEEE Winter Conference on Applications of Computer Vision (WACV 2018), Lake Tahoe, CA, USA}, pages={9}, year={2018} }
Dataset
The annotations can be downloaded from here: Dataset
The annotations are provided as EAF Files, which can be opened in ELAN annotation tool (available at https://tla.mpi.nl/tools/tla-tools/elan/download/). The links to the youtube videos are provided in supplementary (will be required to view the annotations with the video).
We will be releasing the features soon!