Learning Individual Speaking Styles for Accurate Lip to Speech Synthesis
IIIT Hyderabad IIT Kanpur
CVPR 2020
Please click here to redirect to watch our video in Youtube.
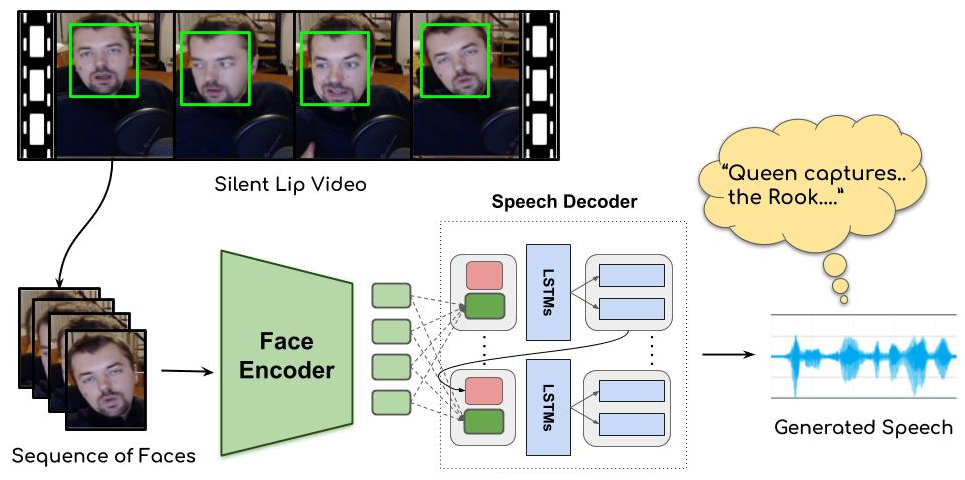
In this work, we propose a sequence-to-sequence architecture for accurate speech generation from silent lip videos in unconstrained settings for the first time. The text in the bubble is manually transcribed and is shown for presentation purposes.
Abstract
Humans involuntarily tend to infer parts of the conversation from lip movements when the speech is absent or corrupted by external noise. In this work, we explore the task of lip to speech synthesis, i.e., learning to generate natural speech given only the lip movements of a speaker. Acknowledging the importance of contextual and speaker-specific cues for accurate lip reading, we take a different path from existing works. We focus on learning accurate lip sequences to speech mappings for individual speakers in unconstrained, large vocabulary settings. To this end, we collect and release a large-scale benchmark dataset, the first of its kind, specifically to train and evaluate the single-speaker lip to speech task in natural settings. We propose an approach to achieve accurate, natural lip to speech synthesis in such unconstrained scenarios for the first time. Extensive evaluation using quantitative, qualitative metrics and human evaluation shows that our method is almost twice as intelligible as previous works in this space.
Paper
Live Demo
Learning Individual Speaking Styles for Accurate Lip to Speech Synthesis (CVPR, 2020) : Please click here to redirect to Youtube.
Dataset

Our dataset contains lectures and chess commentary as of now.
We introduce a new benchmark dataset for unconstrained lip to speech synthesis that is tailored towards exploring the following line of thought: How accurately can we infer an individual’s speech style and content from his/her lip movements? To create this dataset, we collect a total of about 175 hours of talking face videos across 6 speakers. Our dataset is far more unconstrained and natural than older datasets like the GRID corpus and TIMIT dataset. All the corpuses are compared in the table given below.
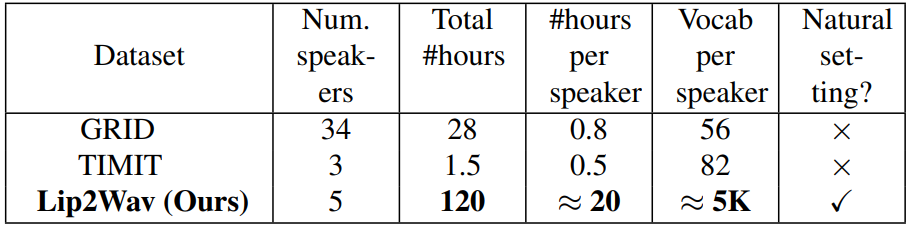
Comparison of our dataset with other datasets which has been used earlier for video-to-speech generation
To access the dataset please click this link or the link given near the top of the page. We release the youtube ids of the videos used. In case, the videos are not present in Youtube, please contact us for an alternate link.
Architecture
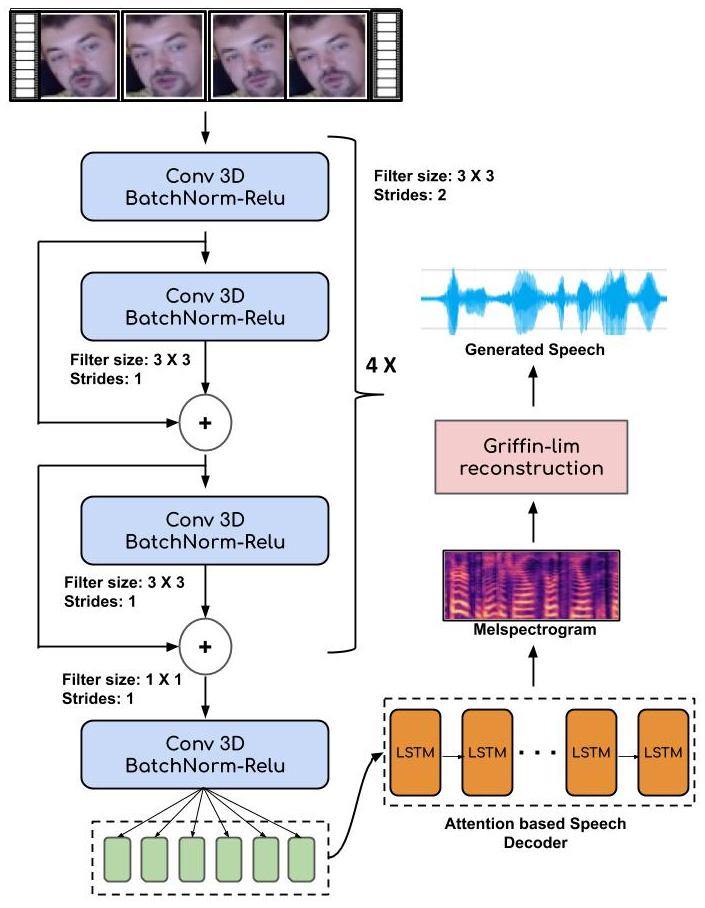
Architecture for generating speech from lip movements
Our network consists of a spatio-temporal encoder and a attention based decoder. The spatio-temporal encoder takes multiple T frames as input and passes through a 3D CNN based encoder. We feed the output from 3D CNN based encoder to a attention based speech decoder to generate melspectrograms following the seq-to-seq paradigm. For more information about our model and different design choices we make please go through our paper.
Contact
- Prajwal K R - This email address is being protected from spambots. You need JavaScript enabled to view it.
- Rudrabha Mukhopadhyay - This email address is being protected from spambots. You need JavaScript enabled to view it.