Quo Vadis, Skeleton Action Recognition ?
Abstract
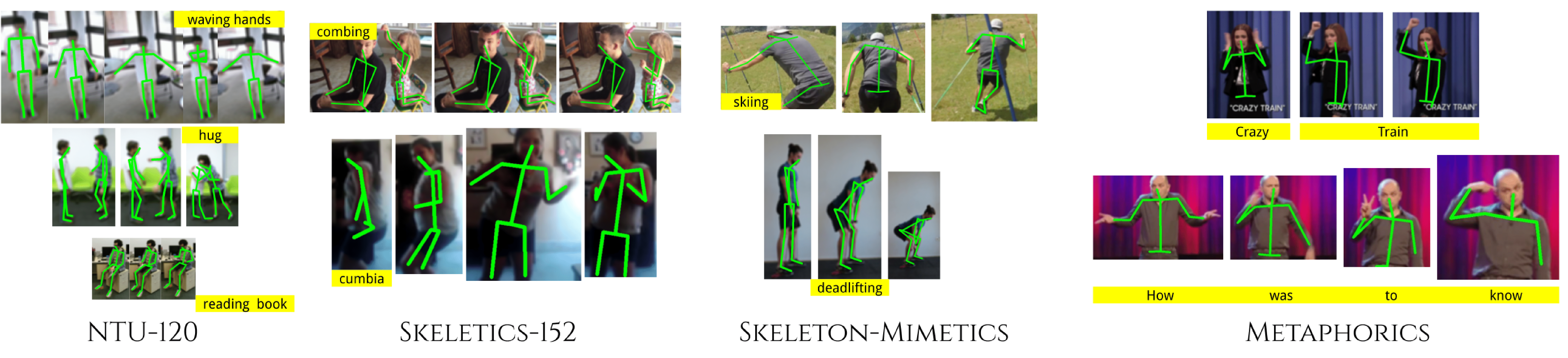
To access the code and paper click here
To access the code and paper click here
Owing to the overparametrized nature and high memory requirements of classical DNNs, there has been a renewed interest in network sparsification. Is it possible to prune a network at initialization (prior to training) while maintaining rich connectivity, and also ensure faster convergence? We attempt to answer this question by emulating the pattern of neural connections in the brain.
Figure: After a preliminary pruning step, N2NSkip connections are added to the pruned network while maintaining the overall sparsity of the network.
The over-parametrized nature of Deep Neural Networks (DNNs) leads to considerable hindrances during deployment on low-end devices with time and space constraints. Network pruning strategies that sparsify DNNs using iterative prune-train schemes are often computationally expensive. As a result, techniques that prune at initialization, prior to training, have become increasingly popular. In this work, we propose neuron-to-neuron skip (N2NSkip) connections, which act as sparse weighted skip connections, to enhance the overall connectivity of pruned DNNs. Following a preliminary pruning step, N2NSkip connections are randomly added between individual neurons/channels of the pruned network, while maintaining the overall sparsity of the network. We demonstrate that introducing N2NSkip connections in pruned networks enables significantly superior performance, especially at high sparsity levels, as compared to pruned networks without N2NSkip connections. Additionally, we present a heat diffusion-based connectivity analysis to quantitatively determine the connectivity of the pruned network with respect to the reference network. We evaluate the efficacy of our approach on two different preliminary pruning methods which prune at initialization, and consistently obtain superior performance by exploiting the enhanced connectivity resulting from N2NSkip connections.
In this work, inspired by the pattern of skip connections in the brain, we propose sparse, learnable neuron-to-neuron skip (N2NSkip) connections, which enable faster convergence and superior effective connectivity by improving the overall gradient flow in the pruned network. N2NSkip connections regulate overall gradient flow by learning the relative importance of each gradient signal, which is propagated across non-consecutive layers, thereby enabling efficient training of networks pruned at initialization (prior to training). This is in contrast with conventional skip connections, where gradient signals are merely propagated to previous layers. We explore the robustness and generalizability of N2NSkip connections to different preliminary pruning methods and consistently achieve superior test accuracy and higher overall connectivity. Additionally, our work also explores the concept of connectivity in deep neural networks through the lens of heat diffusion in undirected acyclic graphs. We propose to quantitatively measure and compare the relative connectivity of pruned networks with respect to the reference network by computing the Frobenius norm of their heat diffusion signatures at saturation.
Figure: As opposed to conventional skip connections, N2NSkip connections introduce skip connections between non-consecutive layers of the network, and are parametrized by sparse learnable weights.
Considering each network as an undirected graph, we construct an n × n adjacency matrix, where n is the total number of neurons in the MLP. To verify the enhanced connectivity resulting from N2NSkip connections, we compare the heat diffusion signature of the pruned adjacency matrices with the heat diffusion signature of the reference network.
Figure: Binary adjacency matrices for (a) Reference network (MLP) (b) Pruned network at a compression of 5x (randomized pruning) (c) N2NSkip network at a compression of 5x (10% N2NSkip connections + 10% sequential connections).
Test Accuracy of pruned ResNet50 and VGG19 on CIFAR-10 and CIFAR-100 with either RP or CSP as the preliminary pruning step. The addition of N2NSkip connections leads to a significant increase in test accuracy. Additionally, there is a larger increase in accuracy at network densities of 5% and 2%, as compared to 10%. This observation is consistent for both N2NSkip-RP and N2NSkip-CSP, which indicates that N2NSkip connections can be used as a powerful tool to enhance the performance of pruned networks at high compression rates.
To access the code and paper click here
If you find our work useful in your research, please consider citing:
@InProceedings{Dwivedi_2020_CVPR_Workshops, author = {Dwivedi, Agam and Saluja, Rohit and Kiran Sarvadevabhatla, Ravi}, title = {An OCR for Classical Indic Documents Containing Arbitrarily Long Words}, booktitle = {The IEEE/CVF Conference on Computer Vision and Pattern Recognition (CVPR) Workshops}, month = {June}, year = {2020} }
Our pipeline consists of 3 parts - each sub-folder in this repo containts code for each:
How to use each is explained in corresponding sub-folder
Please find GitHub Project Page
If you find our work useful in your research, please consider citing:
@article{ puligilla2020topo, author = { Puligilla, Sai Shubodh and Tourani, Satyajit and Vaidya, Tushar and Singh Parihar, Udit and Sarvadevabhatla, Ravi Kiran and Krishna, Madhava }, title = { Topological Mapping for Manhattan-Like Repetitive Environments }, journal = { ICRA }, year = { 2020 }, }
We propose a novel approach that achieves significantly more accurate lip-synchronization (A) in dynamic, unconstrained talking face videos. In contrast, we can see that the corresponding lip shapes generated by the current best model (B) is out-of-sync with the spoken utterances (shown at the bottom).
In this work, we investigate the problem of lip-syncing a talking face video of an arbitrary identity to match a target speech segment. Current works excel at producing accurate lip movements on a static image or on videos of specific people seen during the training phase. However, they fail to accurately morph the actual lip movements of arbitrary identities in dynamic, unconstrained talking face videos, resulting in significant parts of the video being out-of-sync with the newly chosen audio. We identify key reasons pertaining to this and hence resolve them by learning from a powerful lip-sync discriminator. Next, we propose new, rigorous evaluation benchmarks and metrics to specifically measure the accuracy of lip synchronization in unconstrained videos. Extensive quantitative and human evaluations on our challenging benchmarks show that the lip-sync accuracy of the videos generated using our Wav2Lip model is almost as good as real synced videos. We clearly demonstrate the substantial impact of our Wav2Lip model in our publicly available demo video. We also open-source our code, models, and evaluation benchmarks to promote future research efforts in this space.
Prajwal Renukanand*, Rudrabha Mukhopadhyay*, Vinay Namboodiri and C.V. Jawahar
A Lip Sync Expert Is All You Need for Speech to Lip Generation In the Wild, ACM Multimedia, 2020 .
[PDF] | [BibTeX]
Please click here for the live demo : https://www.youtube.com/embed/0fXaDCZNOJc
Architecture for generating speech from lip movements
Our approach generates accurate lip-sync by learning from an ``already well-trained lip-sync expert". Unlike previous works that employ only a reconstruction loss or train a discriminator in a GAN setup, we use a pre-trained discriminator that is already quite accurate at detecting lip-sync errors. We show that fine-tuning it further on the noisy generated faces hampers the discriminator's ability to measure lip-sync, thus also affecting the generated lip shapes.
To ensure fair use, we strongly require that any result created using this our algorithm must unambiguously present itself as synthetic and that it is generated using the Wav2Lip model. In addition, to the strong positive applications of this work, our intention to completely open-source our work is that it can simultaneously also encourage efforts in detecting manipulated video content and their misuse. We believe that Wav2Lip can enable several positive applications and also encourage productive discussions and research efforts regarding fair use of synthetic content.