PALMIRA: A Deep Deformable Network for Instance Segmentation of Dense and Uneven Layouts in Handwritten Manuscripts
Abstract
Handwritten documents are often characterized by dense and uneven layout. Despite advances, standard deep network based approaches for semantic layout segmentation are not robust to complex deformations seen across semantic regions. This phenomenon is especially pronounced for the low-resource Indic palm-leaf manuscript domain. To address the issue, we first introduce Indiscapes2, a new large-scale diverse dataset of Indic manuscripts with semantic layout annotations. Indiscapes2 contains documents from four different historical collections and is 150% larger than its predecessor, Indiscapes. We also propose a novel deep network Palmira for robust, deformation-aware instance segmentation of regions in handwritten manuscripts. We also report Hausdorff distance and its variants as a boundary-aware performance measure. Our experiments demonstrate that Palmira provides robust layouts, outperforms strong baseline approaches and ablative variants. We also include qualitative results on Arabic, South-East Asian and Hebrew historical manuscripts to showcase the generalization capability of Palmira.
Architecture
The PALMIRA architecture. The orange blocks in the backbone are deformable convolutions. A closer look at the DefGrid Mask Head and the Backbone alteration is provided below in the Network Architecture section.
Highlights
We introduce Indiscapes2, a collection of handwritten palm-leaf manuscripts which is 150% larger compared to its predecessor Indiscapes and contains two additional annotated collections which greatly increase qualitative diversity. In addition, we introduce a novel deep learning based layout parsing architecture called Palm leaf Manuscript Region Annotator or Palmira in short. Through our experiments, we show that Palmira outperforms the previous approach and strong baselines, qualitatively and quantitatively. Additionally, we demonstrate the general nature of our approach on out-of-dataset historical manuscripts. Complementing the traditional area-centric measures of Intersection-over-Union (IoU) and mean Average Precision (AP), we report the boundary-centric Hausdorff distance and its variants as part of our evaluation approach.
Network Architecture
1) Deformable Convolutions in the Backbone
Deformable Convolutions provide a way to determine suitable local 2D offsets for the default spatial sampling locations
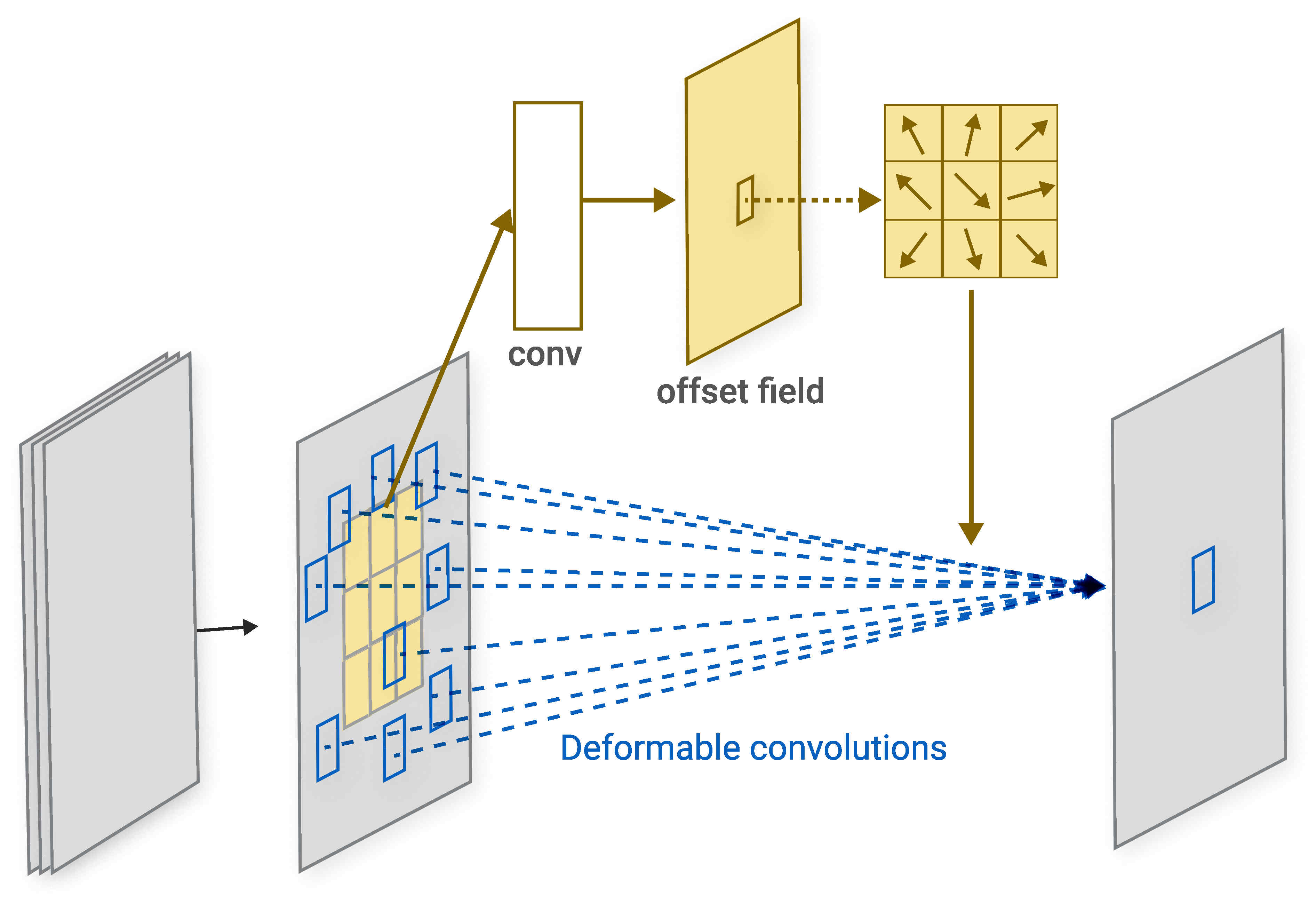
1) Deformable Grid Mask Head
The Deformable Grid Mask Head network is optimized to predict the offsets of the grid vertices such that a subset of edges incident on the vertices form a closed contour which aligns with the region boundary.
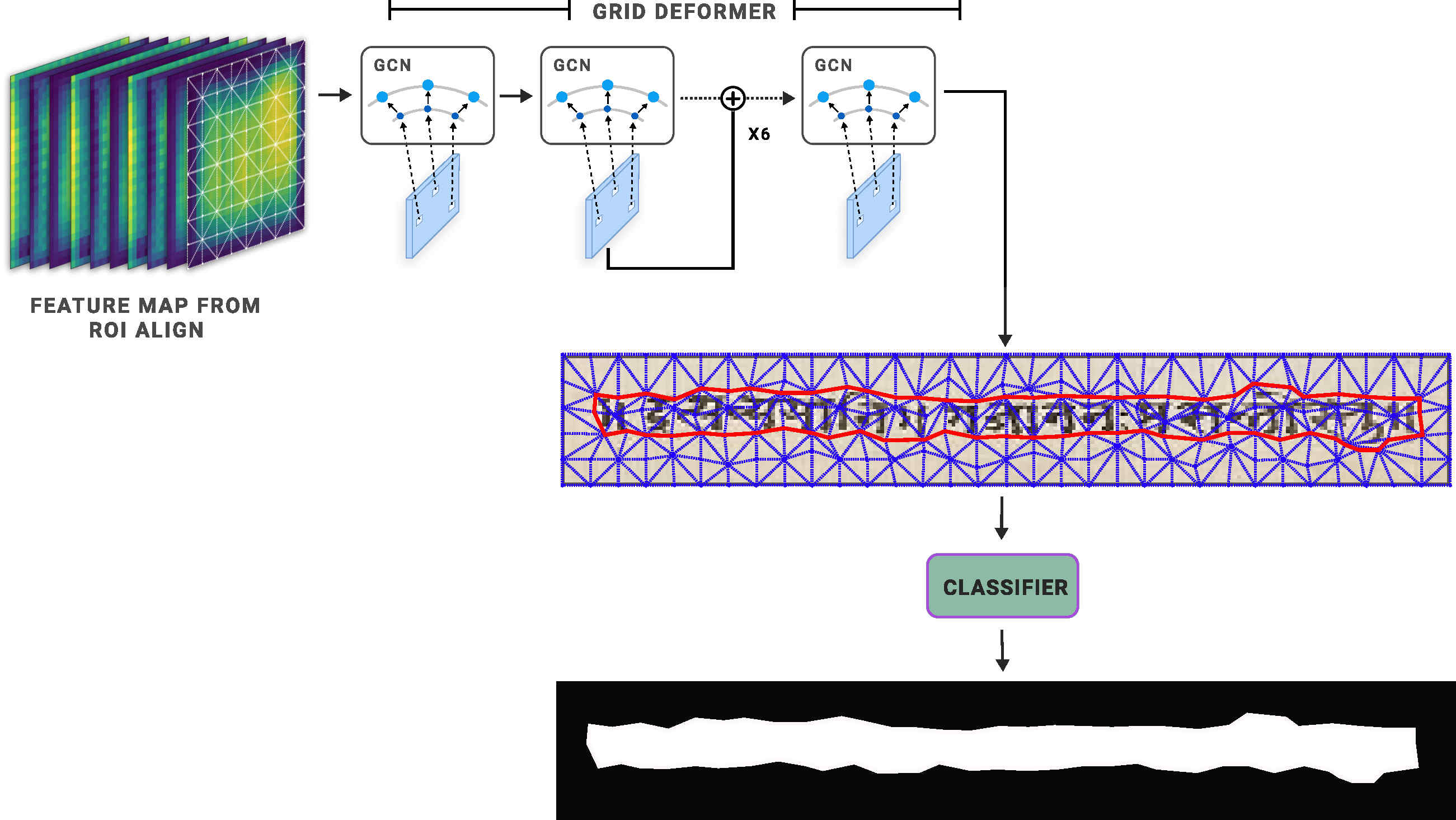
Results
1) Indiscapesv2 Test Set - Document Level
Layout predictions by Palmira on representative test set documents from Indiscapes2 dataset. Note that the colors are used to distinguish region instances. The region category abbreviations are present at corners of the regions.
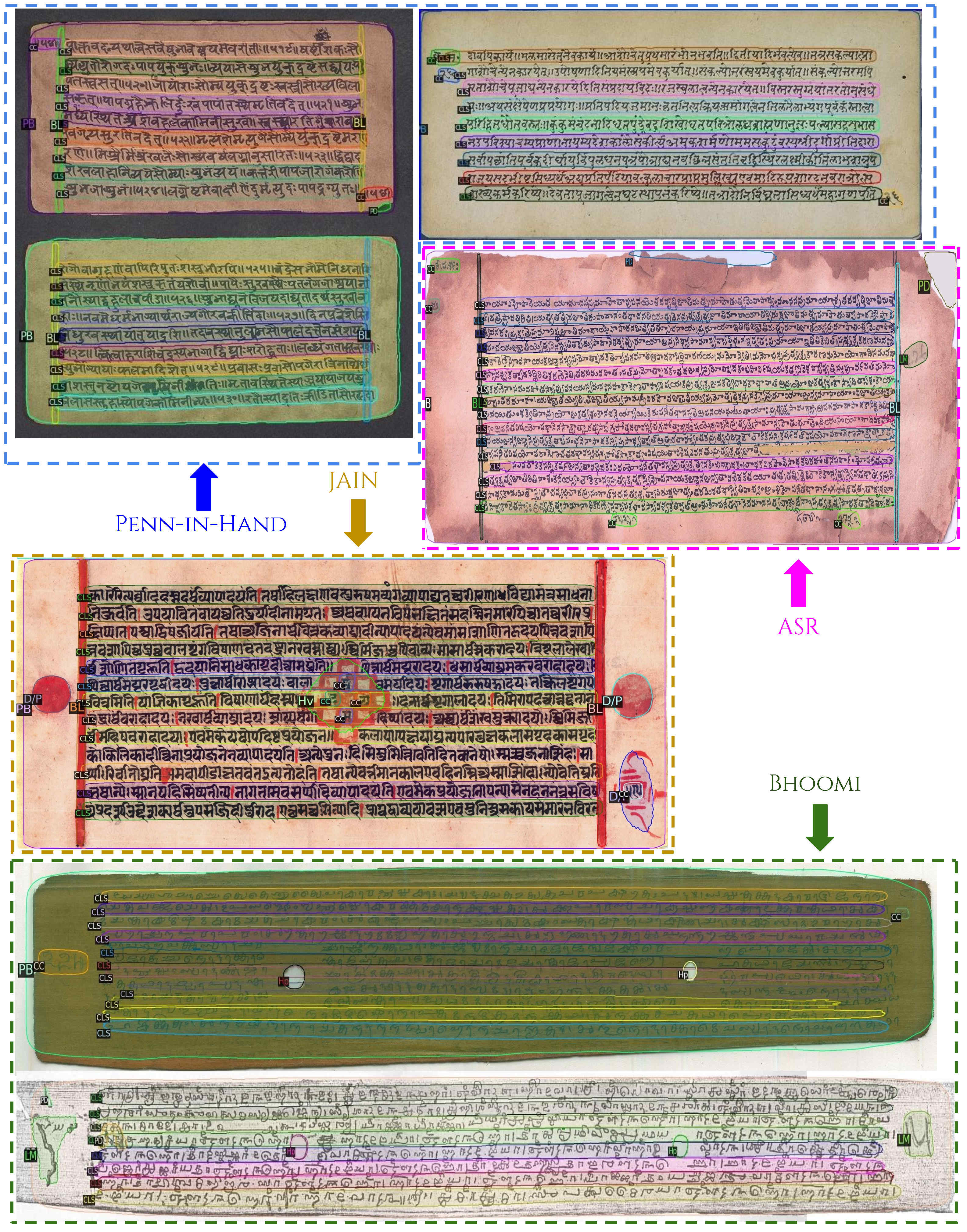
2) Indiscapesv2 Test Set - Region Level Performance
A comparative illustration of region-level performance. Palmira’s predictions are in red. Predictions from the best model among baselines (BoundaryPreserving Mask-RCNN) are in green. Ground-truth boundary is depicted in white.
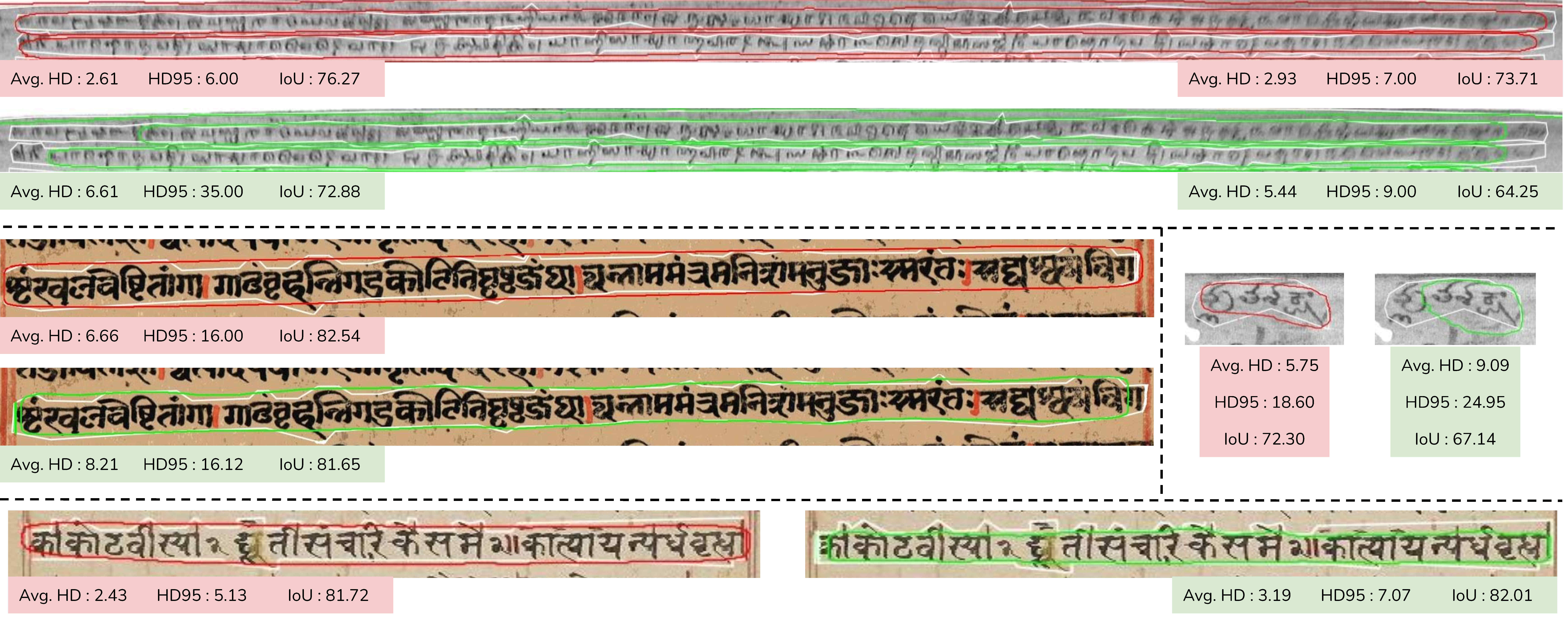
3) Other Documents (Out of Dataset)
Layout predictions by Palmira on out-of-dataset handwritten Manuscripts
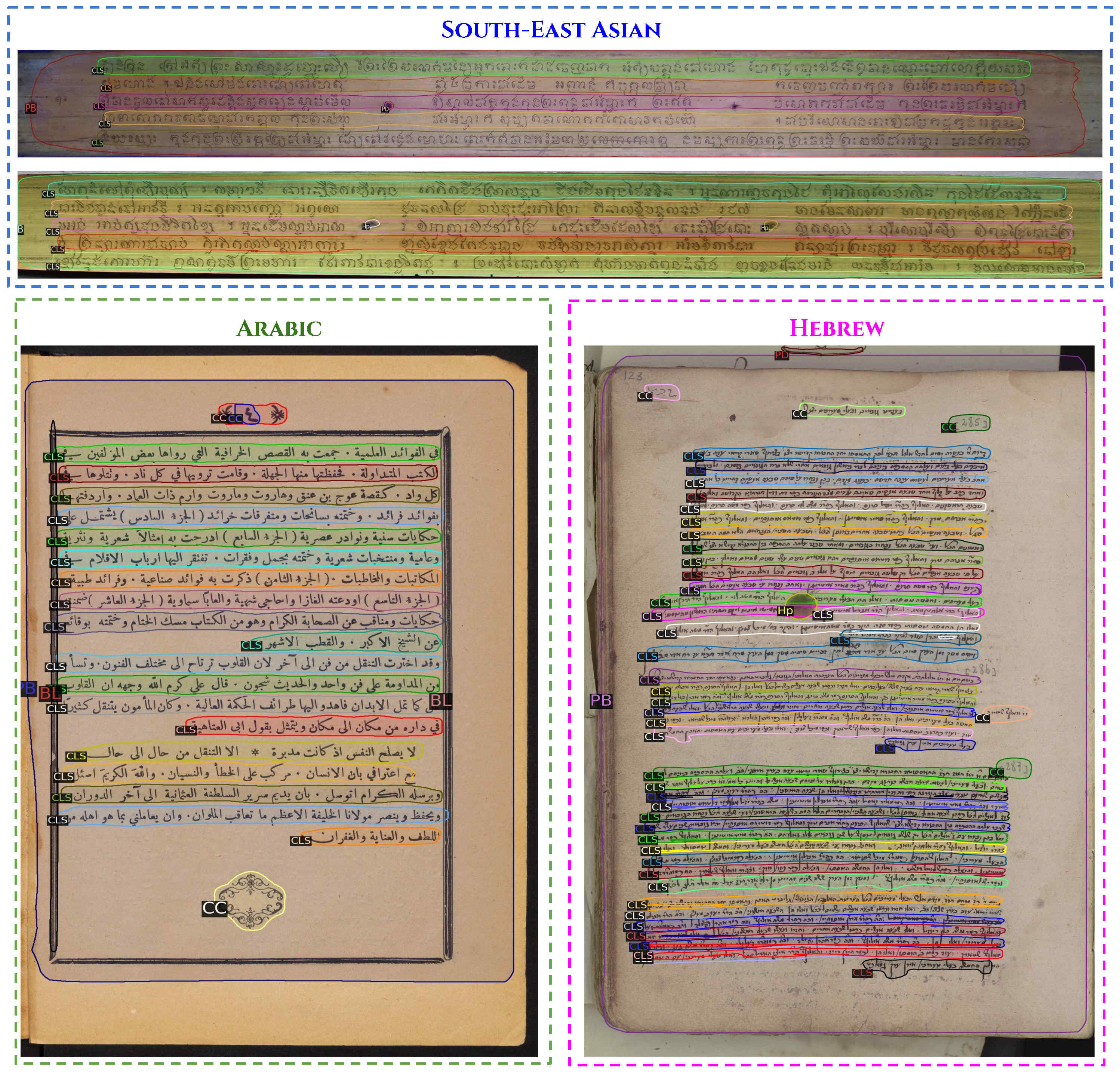
Citation
@inproceedings{sharan2021palmira,
title = {PALMIRA: A Deep Deformable Network for Instance Segmentation of Dense and Uneven Layouts in Handwritten Manuscripts},
author = {Sharan, S P and Aitha, Sowmya and Amandeep, Kumar and Trivedi, Abhishek and Augustine, Aaron and Sarvadevabhatla, Ravi Kiran},
booktitle = {International Conference on Document Analysis and Recognition,
{ICDAR} 2021},
year = {2021},
}
Contact
If you have any question, please contact Dr. Ravi Kiran Sarvadevabhatla at This email address is being protected from spambots. You need JavaScript enabled to view it. .